From Pilots to Production
2024 marks the year that generative AI became a mission-critical imperative for the enterprise. The numbers tell a dramatic story: AI spending1 surged to $13.8 billion this year, more than 6x the $2.3 billion spent in 2023—a clear signal that enterprises are shifting from experimentation to execution, embedding AI at the core of their business strategies.
This spike in spending reflects a wave of organizational optimism; 72% of decision-makers anticipate broader adoption of generative AI tools in the near future. This confidence isn’t just speculative—generative AI tools are already deeply embedded in the daily work of professionals, from programmers to healthcare providers.
Despite this positive outlook and increasing investment, many decision-makers are still figuring out what will and won’t work for their businesses. More than a third of our survey respondents do not have a clear vision for how generative AI will be implemented across their organizations. This doesn’t mean they’re investing without direction; it simply underscores that we’re still in the early stages of a large-scale transformation. Enterprise leaders are just beginning to grasp the profound impact generative AI will have on their organizations.
Last year, our 2023 State of Generative AI in the Enterprise Report captured a period of early AI experimentation. Our 2024 report, expanded to include insights from 600 U.S. enterprise leaders, reveals the trends emerging as organizations shift from pilots to production, making AI an enterprise imperative.
Generative AI Spending Signals Growing Enterprise Commitment
Today, 60% of enterprise generative AI investments come from innovation budgets, reflecting the early stages of generative AI adoption. However, with 40% of generative AI spending sourced from more permanent budgets—58% of which is redirected from existing allocations—businesses are demonstrating a growing commitment to AI transformation.

While foundation model investments still dominate enterprise generative AI spend, the application layer is now growing faster, benefiting from coalescing design patterns at the infrastructure level. Companies are creating substantial value by using these tools to optimize workflows across sectors, paving the way for broader innovation.
In the following sections, we’ll explore two critical dimensions of enterprise generative AI adoption:
- The application layer, where the first breakouts are emerging and greenfield markets are unfolding for startups; and
- The modern AI stack, where the LLM arms race is reshaping the competitive landscape and where specific infrastructure patterns are gaining widespread adoption.
Deep Dive on Applications: The Application Layer Is Heating Up
In 2024, much of the action happened at the application layer. With many architectural design patterns established, app layer companies are leveraging LLMs’ capabilities across domains to unlock new efficiencies and capabilities. Enterprise buyers are seizing the moment, pouring $4.6 billion into generative AI applications in 2024, an almost 8x increase from the $600 million reported last year.
Companies aren’t just spending more; they’re thinking bigger. On average, organizations have identified 10 potential use cases for this transformative technology, signaling broad and ambitious goals. Nearly a quarter (24%) of these are prioritized for near-term implementation, highlighting strong momentum toward practical deployment. This is just the beginning. Most companies are still in the early stages of adoption, with only a few use cases in production, while a third of them are still being prototyped and evaluated (33%).
Inside the Enterprise: Ranking the Most Valuable Use Cases
Despite ongoing experimentation, clear adoption trends point to a subset of use cases already delivering tangible ROI through enhanced productivity or operational efficiency:
- Code copilots lead the charge with 51% adoption, making developers AI’s earliest power users. GitHub Copilot’s rapid ascent to a $300 million revenue run rate validates this trajectory, while emerging tools like Codeium and Cursor are also growing fast. Beyond general coding assistants, enterprises are also purchasing task-specific copilots like Harness’* AI DevOps Engineer and QA Assistant for pipeline generation and test automation, as well as AI agents like All Hands* that are able to perform more end-to-end software development.
- Support chatbots have captured significant usage, with 31% enterprise adoption. These applications deliver reliable, 24/7, knowledge-based support for internal employees and external customers. Aisera,* Decagon, and Sierra’s agents interact directly with end customers, while Observe AI* supports contact center agents with real-time guidance during calls.
- Enterprise search + retrieval and data extraction + transformation (28% and 27%, respectively) reflect a strong drive to unlock and harness the valuable knowledge hidden within data silos scattered across organizations. Solutions like Glean and Sana* connect to emails, messengers, and document stores—enabling unified semantic search across disparate systems and delivering AI-powered knowledge management.
- Meeting summarization ranks fifth in use cases (24% adoption), saving time and boosting productivity by automating note-taking and takeaways. Tools like Fireflies.ai, Otter.ai, and Sana capture and summarize online meetings, while Fathom distills key points from videos. Eleos Health* applies this innovation to healthcare, automating hours of documentation and integrating directly with EHRs so that healthcare providers can focus on patient care.
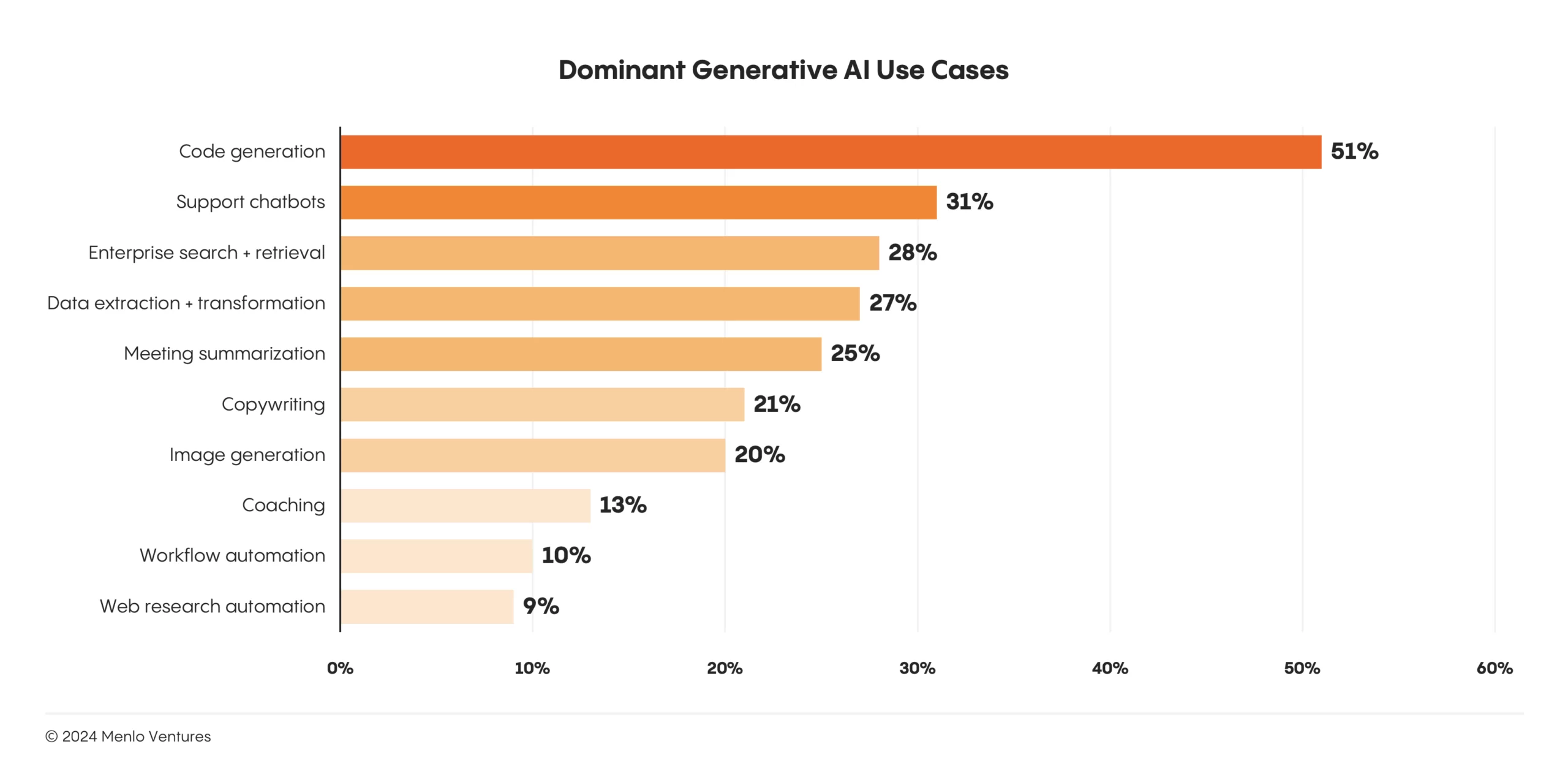
Agents and Automation: AI Takes the Wheel
Current implementation patterns reveal a preference for augmenting human workflows over full automation. But we’re just now approaching a transition to more autonomous solutions. Early examples of AI-powered agents capable of managing complex, end-to-end processes independently are emerging across industries. Pioneers like Forge and Sema4 in financial back office workflows, as well as Clay’s go-to-market tool, demonstrate how fully autonomous generative AI systems can transform traditionally human-led sectors, pointing towards a future “Services-as-Software” era, where AI-driven solutions offer the capabilities of traditional service providers but operate entirely through software.
Build vs. Buy? A Case-by-Case Approach
When deciding to build or buy, companies reveal a near-even split: 47% of solutions are developed in-house, while 53% are sourced from vendors. This is a noticeable shift from 2023, when we reported that 80% of enterprises relied on third-party generative AI software—indicating an increasing confidence and ability for many enterprises to stand up their own internal AI tools rather than rely primarily on external vendors.
The Long Game: Enterprises Prioritize Value Over Quick Wins in Generative AI Adoption
When selecting generative AI applications, enterprises have clear priorities: Return on investment and industry-specific customization matter most when selecting new tools. Surprisingly, price isn’t a major issue; just 1% of the enterprise leaders we surveyed mentioned price as a selection concern. Buyers are playing the long game: They are far more focused on tools that can deliver measurable value (30%) and that understand the unique context of their work (26%) over those offering the lowest price tag (1%).
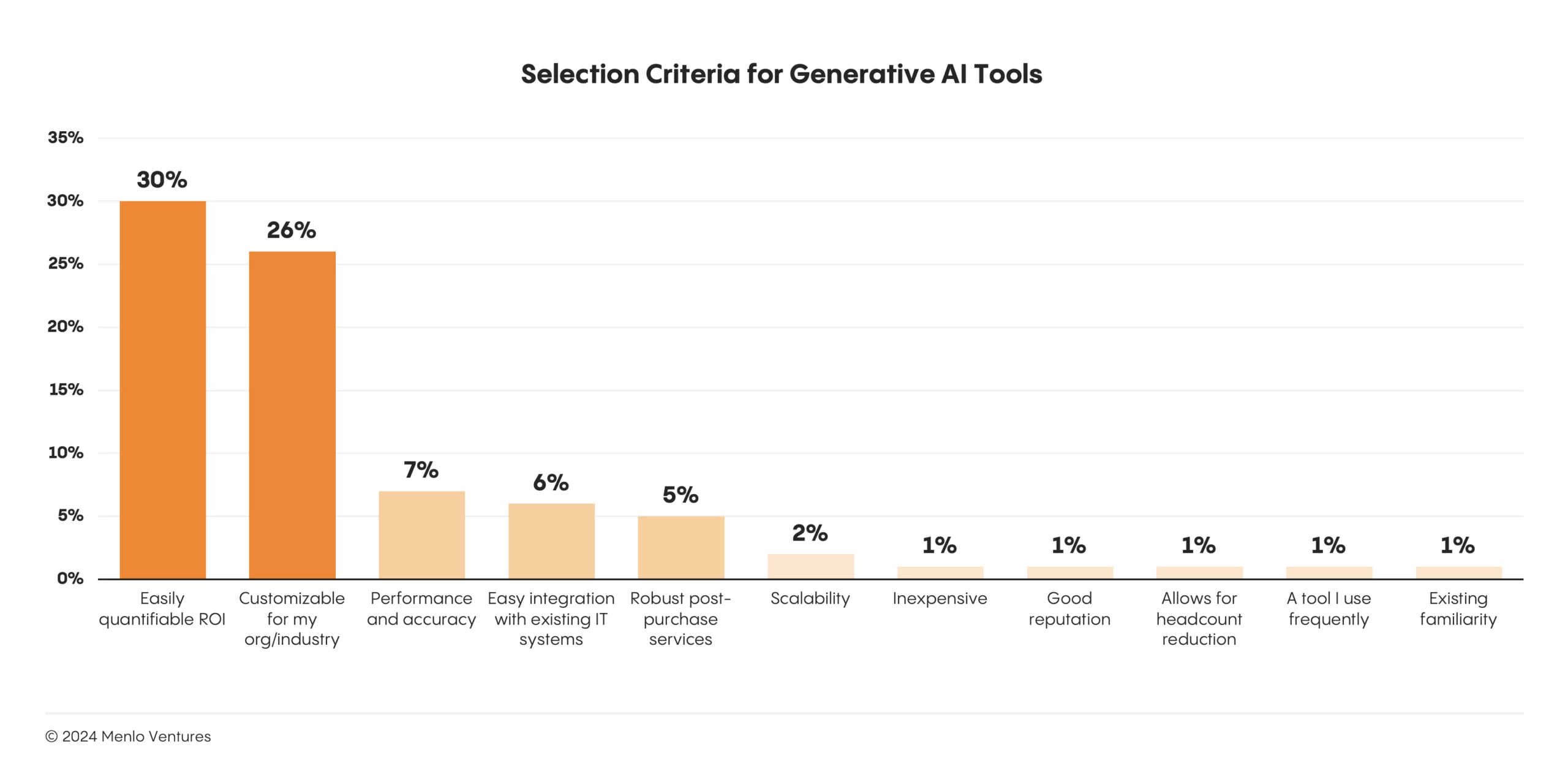
Though businesses are doing their diligence on ROI and customization, they may miss crucial pieces of the implementation puzzle. Often, organizations discover too late that they’ve underestimated the importance of technical integration, ongoing support, and scalability. It’s a bit like buying a car based solely on fuel efficiency, only to realize later that service availability and ease of maintenance are just as critical over the long haul.
When AI pilots stutter or stall, it’s often due to challenges not adequately considered during the selection process. Although buyers aren’t checking price tags, implementation costs, cited in 26% of failed pilots, frequently catch them off guard. Data privacy hurdles (21%) and disappointing return on investment (ROI) (18%) also throw pilots off course. Technical issues, especially around hallucinations (15%), round out the top reasons for failure. Proactively addressing these potential pitfalls during the planning and selection stages can increase the likelihood of a successful implementation.
Bolt-on vs. Breakthrough: Incumbents More Vulnerable to Startup Disruption
Last year, incumbents dominated the enterprise market with a “bolt-on” strategy that layered generative AI capabilities onto existing products. We predicted startups would increasingly gain ground, and this year’s data validates our thinking: While 64% of customers still prefer buying from established vendors, citing trust and out-of-the-box functionality, incumbent dominance is starting to show cracks. Our data reveals a growing dissatisfaction: 18% of decision-makers express disappointment with incumbent offerings; while 40% question whether their current solutions truly meet their needs, signaling an opportunity for innovative startups to step in and stake their claim.
Department-by-Department Transformation
What’s striking about generative AI adoption today isn’t just the scale—it’s the scope. This year, generative AI budgets flowed to every department.
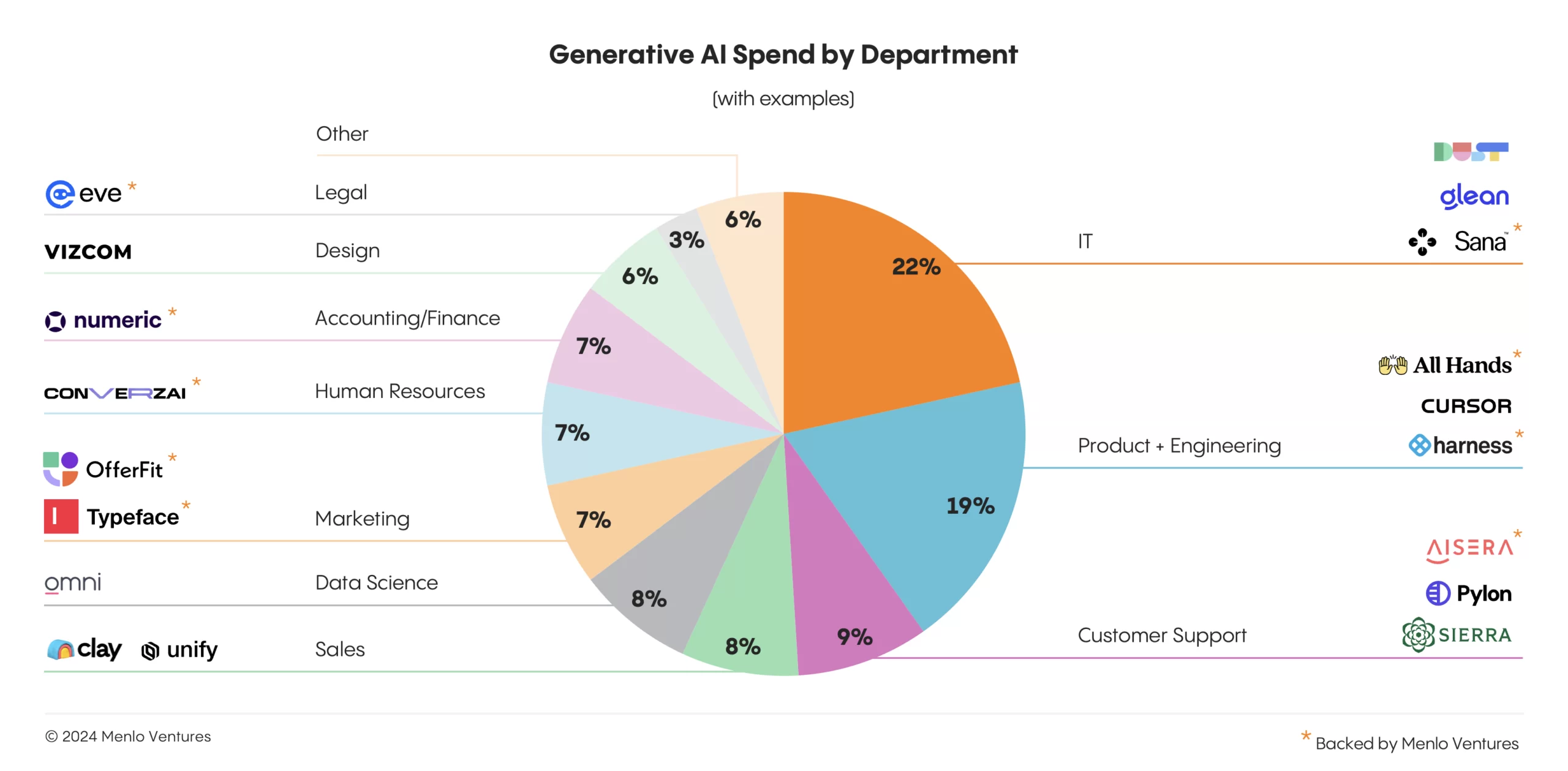
It’s no surprise that technical departments command the largest share of spending, with IT (22%), Product + Engineering (19%), and Data Science (8%) together accounting for nearly half of all enterprise generative AI investments. The remaining budget is distributed across customer-facing functions like Support (9%), Sales (8%), and Marketing (7%), back-office teams including HR and Finance (7% each), and smaller departments like Design (6%) and Legal (3%).
The Rise of Vertical AI Applications
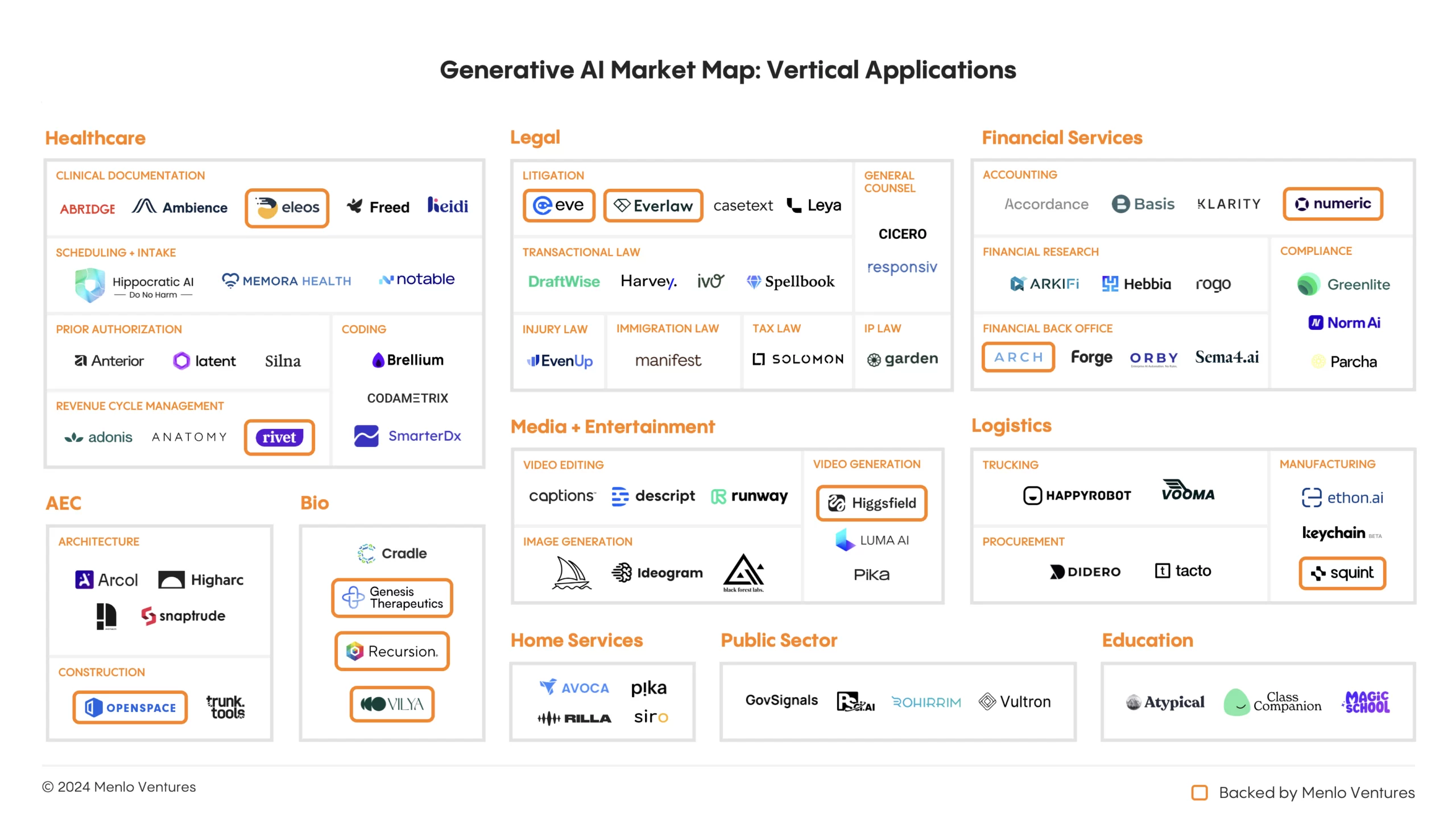
The first generative AI applications were horizontal solutions for text and image generation, but 2024 saw more and more applications finding traction in applying LLMs’ new capabilities to highly domain-specific, verticalized workflows. The following verticals are leading adoption:
- Healthcare: Traditionally slow to adopt tech, healthcare is now leading generative AI adoption with $500 million in enterprise spend. Ambient scribes like Abridge, Ambience, Heidi, and Eleos Health are becoming staples of doctors’ offices, while automation solutions are emerging across the clinical lifecycle—from triage and intake (e.g., Notable) to coding (e.g., SmarterDx, Codametrix) and revenue cycle management (e.g., Adonis, Rivet*).
- Legal: Historically resistant to tech, the legal industry ($350 million in enterprise AI spend) is now embracing generative AI to manage massive amounts of unstructured data and automate complex, pattern-based workflows. The field broadly divides into litigation and transactional law, with numerous subspecialties. Rooted in litigation, Everlaw* focuses on legal holds, e-discovery, and trial preparation, while Harvey and Spellbook are advancing AI in transactional law with solutions for contract review, legal research, and M&A. Specific practice areas are also targeted AI innovations: EvenUp focuses on injury law, Garden on patents and intellectual property, Manifest on immigration and employment law, while Eve* is re-inventing plaintiff casework from client intake to resolution.
- Financial Services: With its complex data, strict regulations, and critical workflows, financial services ($100 million in enterprise AI spend) are primed for AI transformation. Startups like Numeric* and Klarity are revolutionizing accounting, while Arkifi and Rogo accelerate financial research through advanced data extraction. Arch* is using AI to disrupt back-office processes for RIAs and investment funds. Orby and Sema4 are broader horizontal solutions starting with reconciliation and reporting, while Greenlite and Norm AI deliver real-time compliance monitoring to keep pace with evolving regulations.
- Media and entertainment: From Hollywood screens to creators’ smartphones, generative AI is reshaping media and entertainment ($100 million in enterprise AI spend). Tools like Runway are now studio-grade staples, while apps like Captions and Descript empower independent creators. Platforms like Black Forest Labs, Higgsfield,* Ideogram, Midjourney, and Pika push the boundaries of image and video creation for professionals.
Deep Dive: Infrastructure and the Modern AI Stack
After a year of rapid evolution, the modern AI stack stabilized in 2024, with enterprises coalescing around the core building blocks that comprise the runtime architectures of most production AI systems.
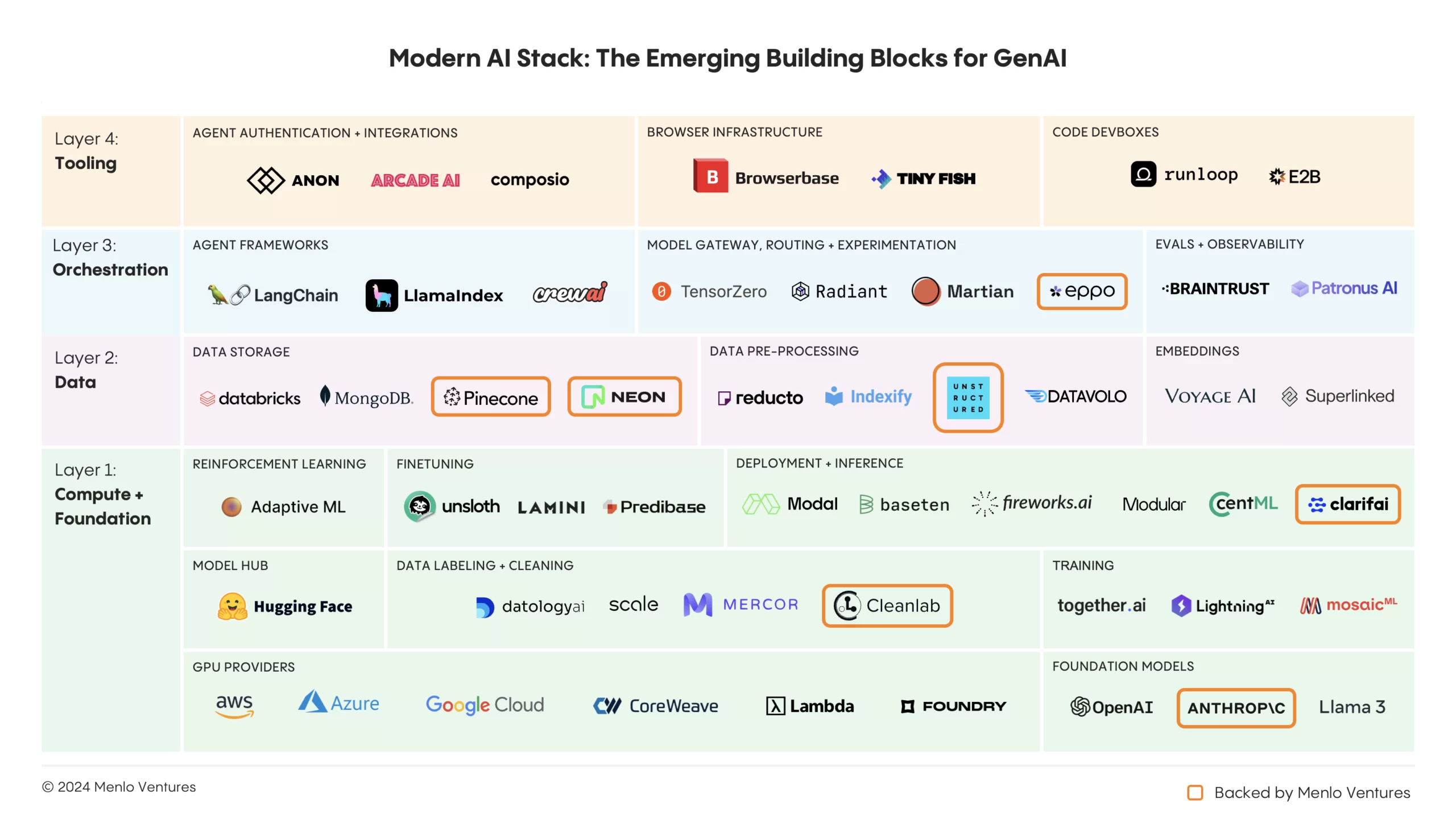
Foundation models still dominate. The LLM layer commands $6.5 billion of enterprise investment. However, through trial and error, enterprises increasingly understand the importance of data scaffolding and integrations in building sophisticated compound AI architectures that can perform reliably in production, not just as one-shot demos.
LLM Trends: Multi-Model Strategies Prevail as OpenAI Cedes Ground to Anthropic
Rather than relying on a single provider, enterprises have adopted a pragmatic, multi-model approach. Our research shows organizations typically deploy three or more foundation models in their AI stacks, routing to different models depending on the use case or results. This strategy extends to the open-versus-closed-source debate, where preferences have remained stable despite heated industry discussions. Closed-source solutions underpin the vast majority of usage with 81% market share, while open-source alternatives (led by Meta’s Llama 3) hold steady at 19%, dropping just one percentage point from 2023.
Among closed-source models, OpenAI’s early mover advantage has eroded somewhat, with enterprise market share dropping from 50% to 34%. The primary beneficiary has been Anthropic,* which doubled its enterprise presence from 12% to 24% as some enterprises switched from GPT-4 to Claude 3.5 Sonnet when the new model became state-of-the-art. When moving to a new LLM, organizations most commonly cite security and safety considerations (46%), price (44%), performance (42%), and expanded capabilities (41%) as motivations.
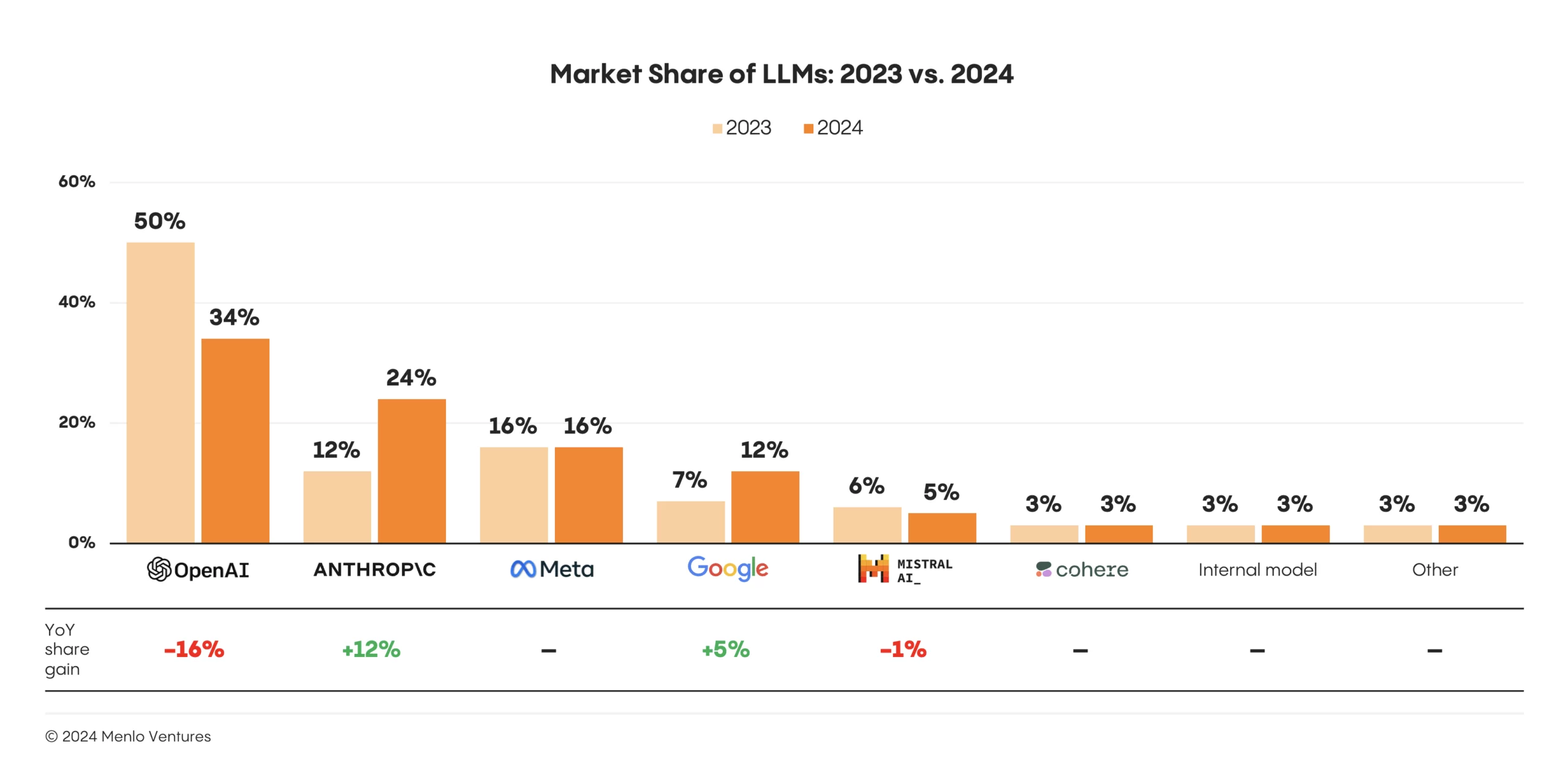
Design Pattern Trends: RAG Gains, Fine Tuning Is Rare, and Agents Break Out
Enterprise AI design patterns—standardized architectures for building efficient, scalable AI systems—are evolving rapidly. RAG (retrieval-augmented generation) now dominates at 51% adoption, a dramatic rise from 31% last year. Meanwhile, fine-tuning—often touted, especially among leading application providers—remains surprisingly rare, with only 9% of production models being fine-tuned.
The year’s biggest breakthrough? Agentic architectures made their debut and already power 12% of implementations.
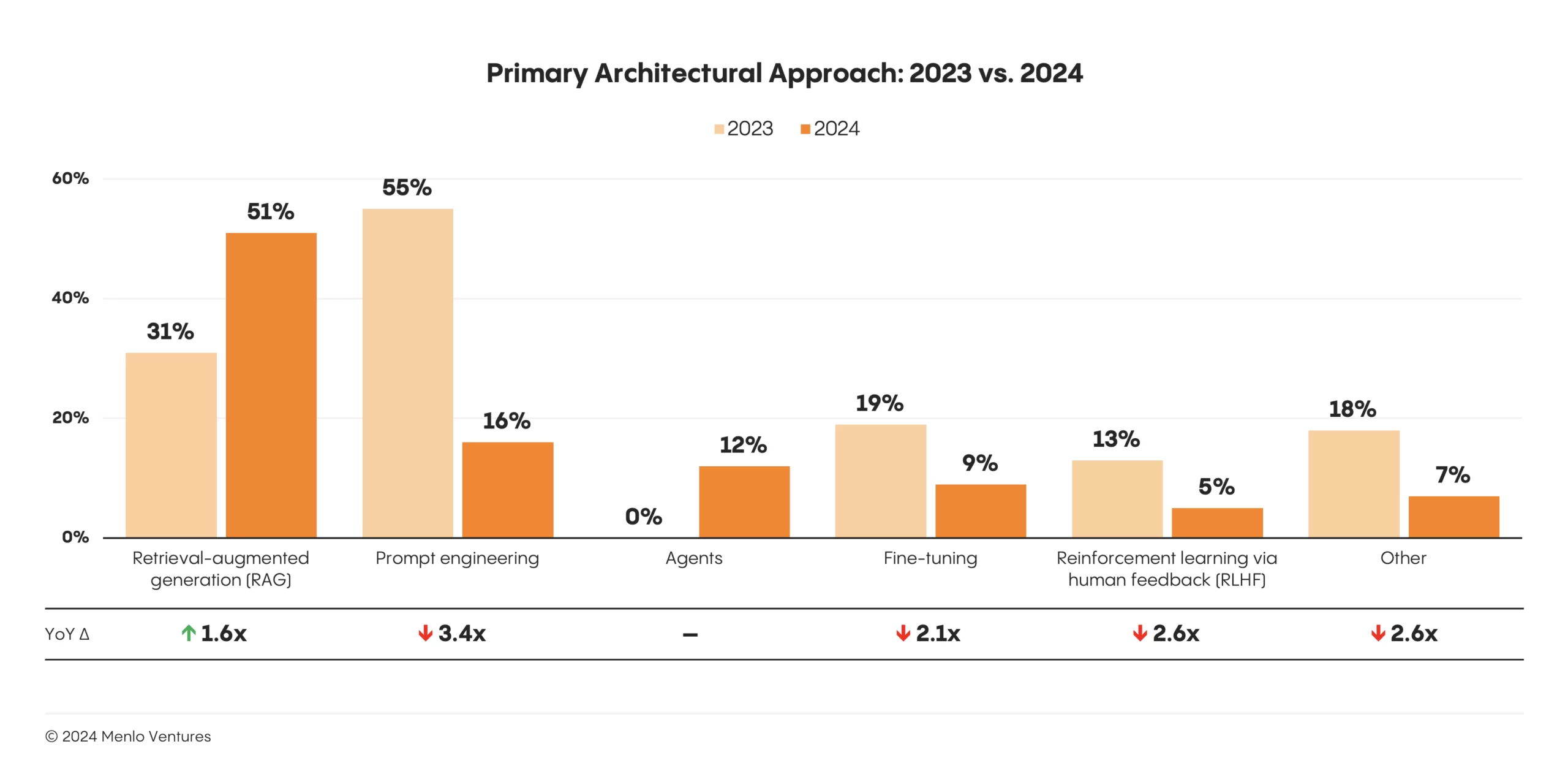
Vector Databases, ETL, and Data Pipelines: The Foundations of RAG
To power RAG, enterprises must store and access relevant query knowledge efficiently. While traditional databases like Postgres (15%) and MongoDB (14%) remain common, AI-first solutions continue to gain ground. Pinecone,* an AI-native vector database, has already captured 18% of the market. A similar shift is happening in data ETL/preparation. Traditional ETL platforms (e.g., Azure Document Intelligence) still account for 28% of deployments, but specialized tools like Unstructured,* designed to handle the nuances of unstructured data in documents such as PDFs and HTML, are carving out their own space, with 16% market share. Across the stack, we see demand for technologies purpose-built to meet the needs of modern AI.
Our Predictions
2024 has been a year of transition and evolution, as the wave of hype we documented in 2023 gave way to real-world implementation. Drawing on the data we’ve shared today and the trends we’ve observed as investors, these are our three predictions for what lies ahead:
- Agents will drive the next wave of transformation.
Agentic automation will drive the next wave of AI transformation, tackling complex, multi-step tasks that go beyond the capabilities of current systems focused on content generation and knowledge retrieval. Platforms like Clay and Forge foreshadow how advanced agents could disrupt the $400 billion software market—and eat into the $10 trillion U.S. services economy. This shift will demand new infrastructure: agent authentication, tool integration platforms, AI browser frameworks, and specialized runtimes for AI-generated code. - David beats Goliath: More incumbents to fall.
ChatGPT’s disruption of Chegg and Stack Overflow this year was a wake-up call: Chegg saw 85% of its market cap vanish, while Stack Overflow’s web traffic halved. Other categories are ripe for disruption. IT outsourcing firms like Cognizant and legacy automation players like UiPath should brace for AI-native challengers moving into their market. Over time, even software giants like Salesforce and Autodesk will face AI-native challengers. - No relief in sight: The AI talent drought intensifies.
We are on the brink of a massive talent drought. The tech industry will encounter severe scarcity as AI systems proliferate and become more sophisticated. This isn’t just a shortage of data scientists—it’s a critical gap in experts who can bridge advanced AI capabilities with domain-specific expertise. The talent pool is already dangerously low. Brace for soaring competition and 2-3x salary premiums for already well-paid AI-skilled enterprise architects becoming the norm. Despite investments in training programs and AI centers of excellence, the gap will outpace these efforts, fueling intense competition for the limited talent needed to power the next wave of AI innovation.
This Is Just the Beginning
AI is paving the way for a new era of transformation driven by cutting-edge AI tools, empowered workforces, and transformative business models that will reshape our economy. We’re witnessing this transformation firsthand across our portfolio:
- AI enables Nobel Prize-winning scientist David Baker, co-founder of Vilya and Xaira Therapeutics, to generate new potential drugs computationally and to predict their structure and function, helping accelerate the development of new lifesaving treatments for patients in need.
- AI enables manufacturers like Continental, Michelin, and Nestlé to capture tribal knowledge and maintain high-standard factory work. Using Squint, they transform instructional materials into immersive augmented reality experiences, providing real-time, step-by-step guidance and verification to enhance safety, reduce operator errors, and ensure consistent throughput.
- AI enables companies to identify top engineering talent faster and more reliably, using CodeSignal to assess technical skills with greater accuracy and efficiency by automating coding assessments, personalizing question difficulty, and detecting plagiarism.
- AI enables Abnormal Security to protect corporations from sophisticated email threats like phishing and business email compromise, preventing up to $4 million in potential losses each year by detecting anomalies and stopping attacks before they cause harm.
- AI empowers Fortune 500 brands across industries to craft personalized, on-brand content at scale with Typeface. With Typeface, brands can quadruple their advertising output, slash production time by half, and save hundreds of hours each month—all while delivering engaging content that resonates with customers.
The team at Menlo Ventures is excited to be part of this transformation, backing founders who are pushing the boundaries of what’s possible with AI. Our portfolio companies—including Abnormal Security, Anthropic, Arch, Cleanlab, Eleos, Genesis Therapeutics, Harness, Higgsfield, Neon, OpenSpace, Pinecone, Recursion, Sana, Squint, Typeface, Unstructured, Vilya, and Xaira—showcase how AI can transform industries and create entirely new tools and businesses that benefit millions. We are getting closer to realizing the potential of the intelligent enterprise. If you’re a founder ready to make your mark, please reach out.
Menlo Ventures is ALL IN on AI. Let’s build the future of AI together.
Data Sources and Methodology
This report summarizes data from a survey of 600 U.S. IT decision-makers at enterprises with 50 or more employees conducted between September 24 and October 8, 2024. Across that foundational data, we overlaid our perspective and insights as active investors in the space.
*Backed by Menlo Ventures
- Generative AI spending includes dollars that went to foundation models, model training + deployment, AI-specific data infrastructure, and new generative AI applications from both startups and incumbents (e.g., Microsoft Copilot, Salesforce Agentforce, Adobe Firefly). Note that this market sizing does not include revenue for chips (e.g., Nvidia), compute (e.g., AWS, GCP, Azure), or AI features (e.g., Intuit Assist). ↩︎
Tim is a partner at Menlo Ventures focused on early-stage investments that speak to his passion for AI/ML, the new data stack, and the next-generation cloud. His background as a technology builder, buyer, and seller informs his investments in companies like Pinecone, Neon, Edge Delta, JuliaHub, TruEra, ClearVector, Squint, and…
A self-described “tall, slightly nerdy product guy,” Joff was previously the Chief Product Officer of Atlassian, responsible for leading its acclaimed portfolio of products, including Jira, Confluence, and Trello. During that time, Joff was named on the “Global CPO 20” list by Products That Count and as a “Top 20…
As an investor at Menlo Ventures, Derek concentrates on identifying investment opportunities across the firm’s thesis areas, covering AI, cloud infrastructure, digital health, and enterprise SaaS. Derek is especially focused on investing in soon-to-be breakout companies at the inflection stage. Derek joined Menlo from Bain & Company where he worked…