For Menlo Ventures’ State of Generative AI in the Enterprise report, we surveyed more than 450 enterprise executives across the U.S. and Europe and spoke to a dozen more to provide a view into generative AI adoption in the enterprise today.
Despite the hype, our research revealed that enterprise investment in generative AI is still surprisingly small compared to other software categories. Most of the value that will be created remains to be seen. While incumbents dominate the market today, we’ve identified three areas of opportunity where startups have the best chances to win.
Below, we will dig into our research, sharing actual budget data and key findings to provide a view into how generative AI is perceived—and prioritized—by enterprise buyers.
Enterprise spend: What do the numbers reveal?
Enterprise investment in generative AI—which we estimate to be $2.5 billion this year—is surprisingly small compared to the enterprise budgets for traditional AI ($70 billion) and cloud software ($400 billion).
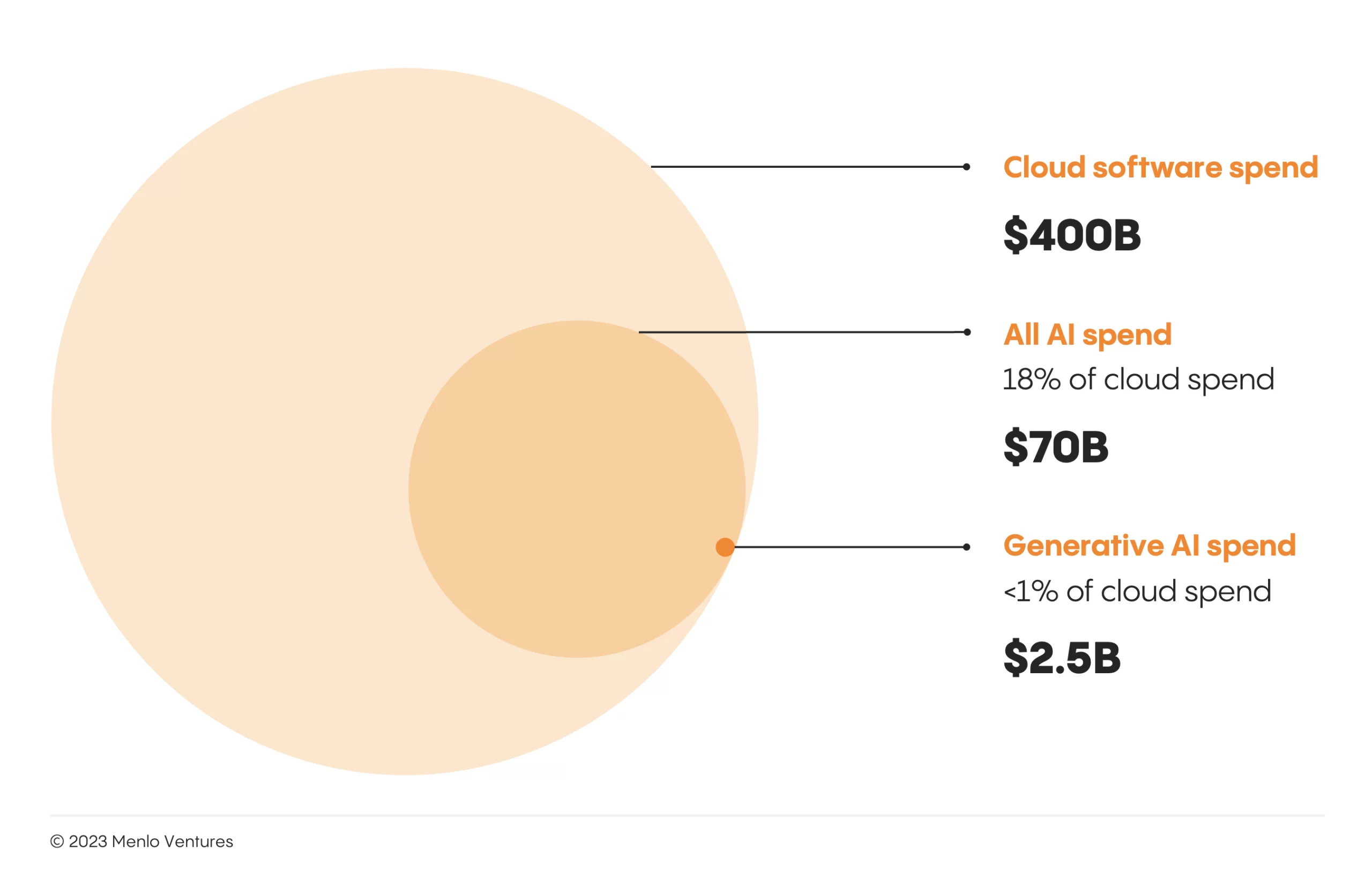
Of course, AI is not new to the enterprise; traditional AI technologies (computer vision, deep learning, etc.) were in use long before generative AI emerged. Half of the enterprises we polled implemented some form of AI, whether into customer-facing products or for internal automation, prior to 2023.
The launch of ChatGPT marked a turning point: AI strategy suddenly became a hot topic in the boardroom. Adoption increased. After five years of stagnation, our survey found:
- The number of enterprises using some form of AI ticked up 7% (from 48% in 2022 to 55% in 2023).
- During that same period, AI spend within enterprises grew by an average of 8%. This ate into total enterprise tech spend, which only grew by 5%.
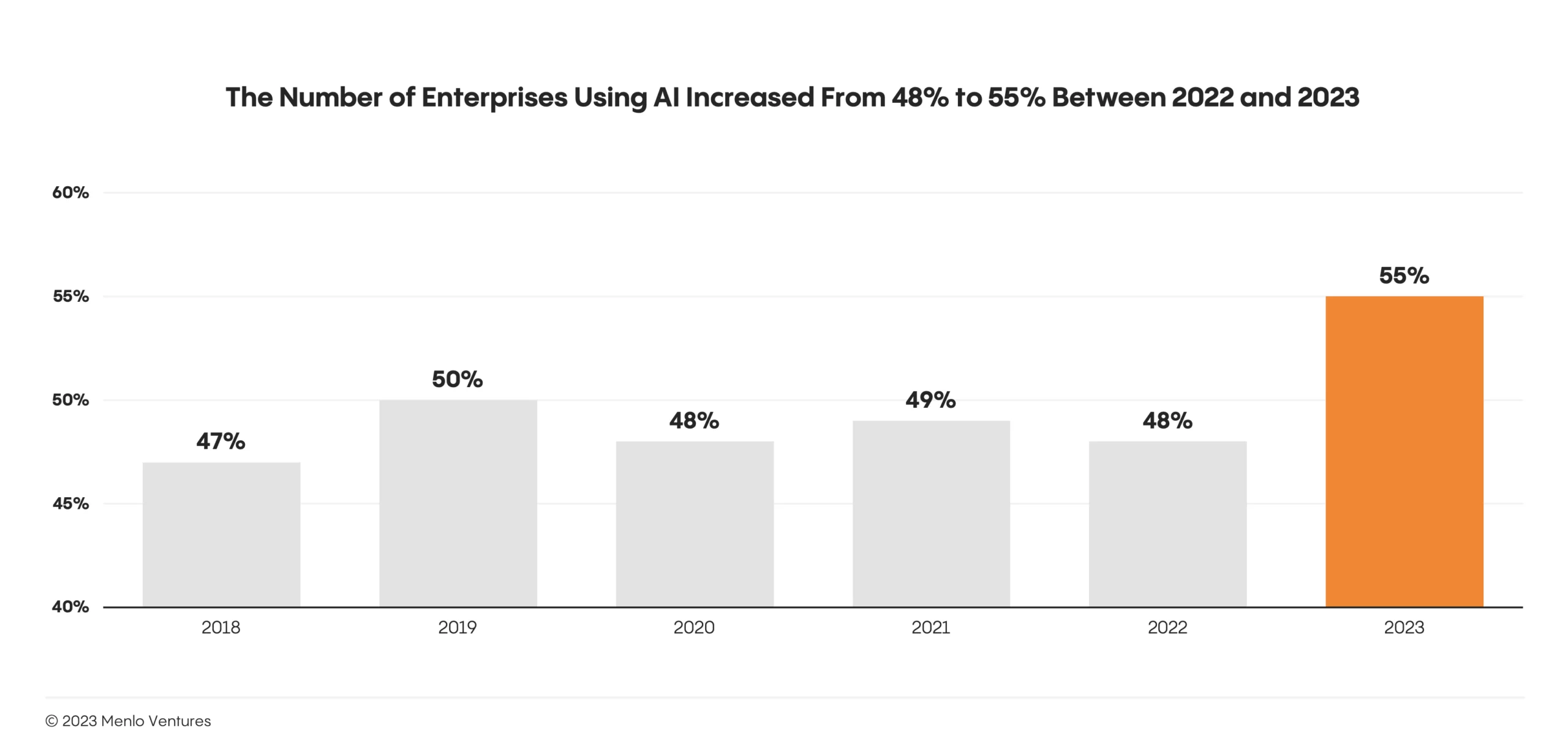
Investments in generative AI contributed to the increase in AI spending. When it comes to build vs. buy, today’s businesses buy; 80% of the survey respondents reported purchasing third-party generative AI software.
Enterprises spent approximately $2.5 billion on generative AI in 2023,1 propelling the rise of tools like GitHub Copilot and Hugging Face (both of which surpassed tens of millions of dollars in revenue). But the market remains nascent. Today, enterprise investment in generative AI still represents less than 1% of all cloud spend.2
More importantly, the generative AI imperative also fueled demand for classical, non-generative AI applications like the data science platform Dataiku and infrastructure provider Databricks. According to our research, traditional AI applications and infrastructure solutions represent more than $70 billion in spend, significantly higher than the dollars dedicated to new LLM-based software and tools and a far more sizable portion of the $400 billion cloud software and infrastructure market.
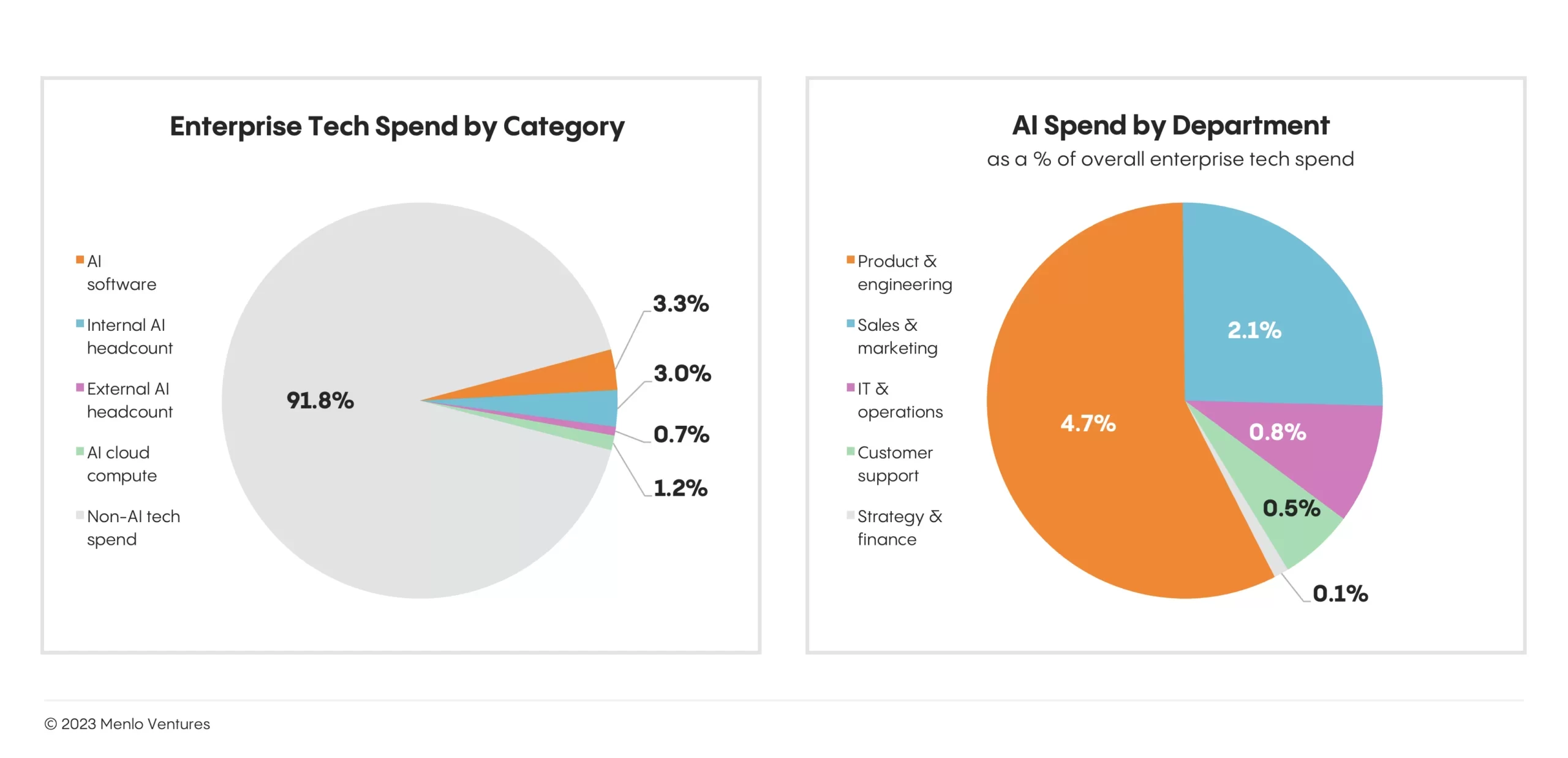
When comparing departmental budgets, we found that product and engineering spend more on AI and generative AI than any other department. In fact, the median enterprise we surveyed spends more on AI for product and engineering (4.7% of all enterprise tech spend) than on all other departments combined (3.5%).
The propensity for product and engineering organizations to build with AI in-house drives those costs. Here, enterprises are investing heavily in AI teams and tech. They are tasking traditional developers and data scientists with building internal infrastructure, and some are hiring additional AI specialists (ML engineers, research scientists, etc.). They are also investing heavily in third-party solutions that are core to the modern AI stack (e.g., databases, data pipelines, and developer tooling).
That said, it’s still early: Today, product and engineering teams drive AI investment, but as solutions evolve to deliver more value, we expect generative AI investment to increase across departments.
Prediction 1: Despite the hype, enterprise adoption of generative AI will be measured, like early cloud adoption
The rise of ChatGPT inspired comparisons between the emergence of generative AI and the introduction of mobile technologies and the internet. But, although consumers quickly—and enthusiastically—embraced generative AI, we expect enterprise AI adoption to be slower, resembling early enterprise adoption of cloud computing.
In the near term, startups may struggle as a result. Many first-wave new entrants are still trying to differentiate themselves, making it hard to gain traction in a market where solutions are plentiful but sellers are hesitant.
In contrast, the early winners who managed to cut through the noise differentiated through technology. For example, Typeface* developed a deeply technical product with an enterprise-wide feedback loop.
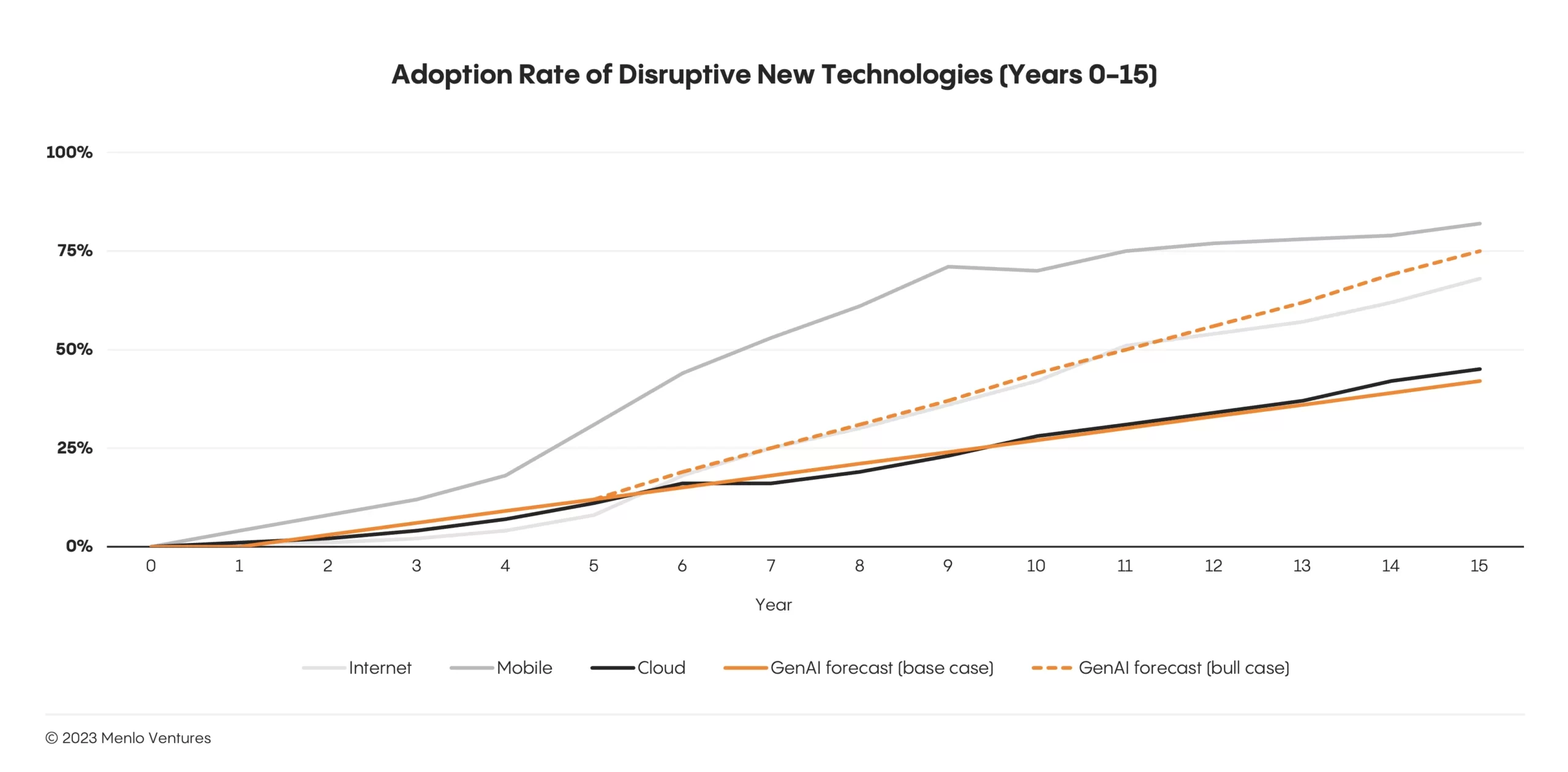
Prediction 2: The market will continue to favor incumbents who embed AI into existing products
The current market favors incumbents who, in contrast to their younger competitors, maintain powerful advantages in scale, distribution, brand, and engineering resources. While competing for generative AI market share, existing players have moved with surprising speed to embrace an “embedded AI strategy”—which, as the name implies, involves embedding AI capabilities into an existing product.
As an example, Microsoft Copilot is an AI companion that spans all of Microsoft’s applications and experiences (e.g., Microsoft 365, Windows 11, Edge, and Bing) to offer AI assistance across almost every aspect of a user’s workflows. According to the company’s CFO, generative tools including Copilot could contribute over $10 billion in revenue over the coming years.4
Tools like Copilot stand in contrast to a newer wave of AI-native solutions that map to existing SaaS categories (departmental, vertical, and horizontal AI applications). These new AI entrants compete in a crowded market against the deep pockets of category leaders. For every AI CRM, there is a Salesforce Einstein; for every AI design tool, a Figma copilot; and for every contact center agent, an Observe.AI*.
We expect the incumbent advantage will hold for the next few years until new and more powerful AI approaches, like agents and multi-step reasoning, become prevalent.
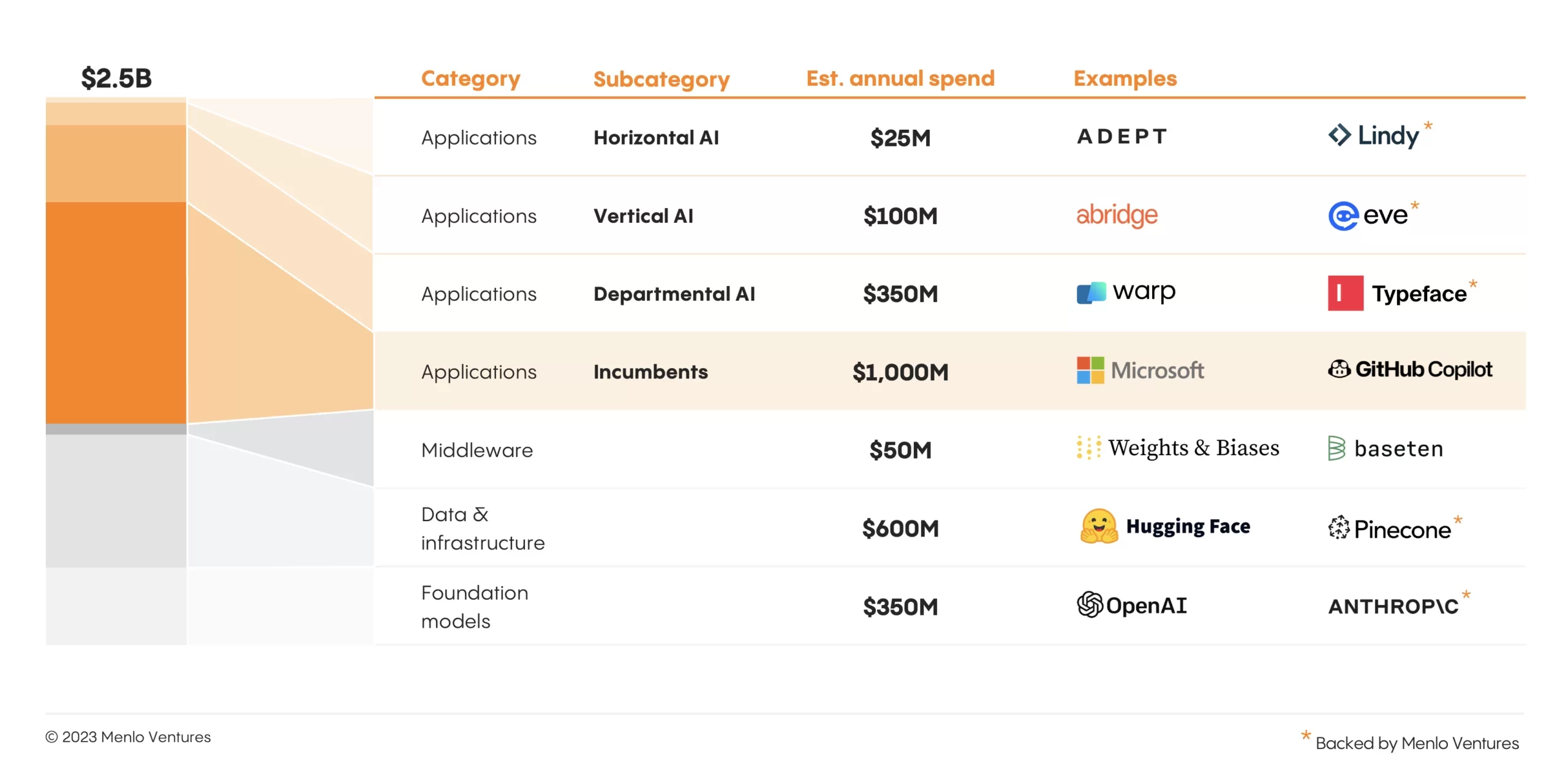
Prediction 3: Powerful context-aware, data-rich workflows will be the key to unlocking enterprise generative AI adoption
The (relatively paltry) $2.5 billion invested in generative AI today indicates that enterprise solutions have yet to deliver on their promise of meaningful transformation. They have failed to create new workflows and behaviors; productivity gains feel limited.
Buyers will remain skeptical until the value is clear. Enterprise execs cite “unproven return on investment” as the most significant barrier to adoption. And on the other side of that coin, enterprise buyers prioritize “performance & accuracy” above all other criteria when making a purchase decision.
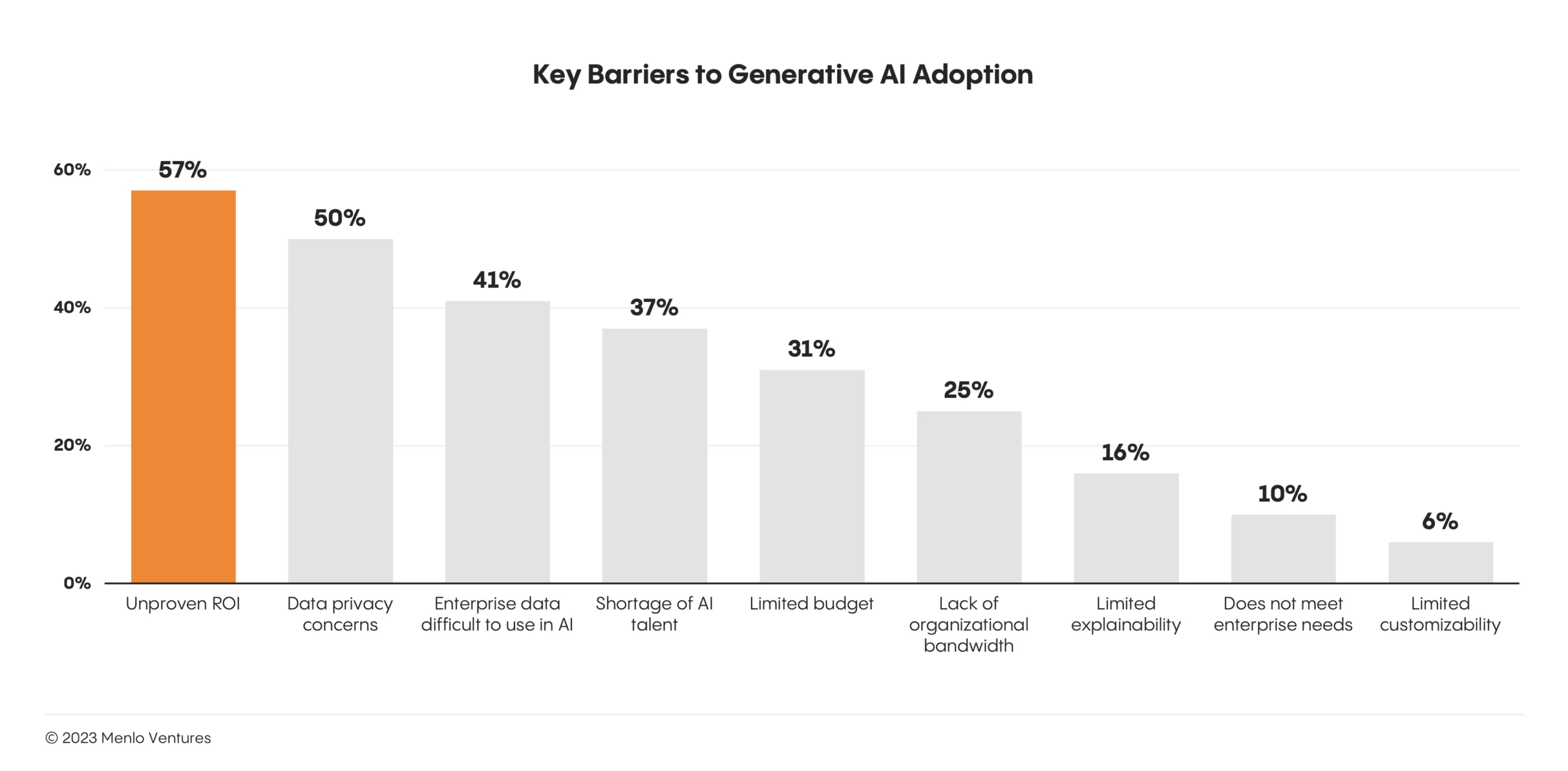
It’s the iron law of the enterprise: Challengers must offer something radically better than the status quo. Enterprise buyers will not be moved by incremental gains in efficiency. AI solutions must demonstrate significant gains in productivity, replace old methodologies, and rewrite workflows in ways that feel entirely novel. For high-potential startups, this means:
- Next-generation reasoning capabilities. Emerging techniques like agent architectures, chain-of-thought, and reflexion will give startups the ability to perform multi-step reasoning and enable more complex tasks than incumbents can easily bolt on to existing products.
- Proprietary data. The next generation of AI natives will be able to incorporate and learn from valuable proprietary data sets—including customer feedback—giving them an edge over the programmatic logic used by many incumbents (e.g., via reinforcement learning or fine-tuning).
- Workflows and enterprise-wide feedback loops. Startups can “close the loop” on many workflows and enable enterprise-wide feedback and workflow optimization in a way completely foreign to existing enterprise workflows (many of which are open-ended daisy chains of various software, data systems, and humans).
This will be the future of enterprise work. Startups that deliver context-aware, data-rich workflows will be the ones that finally unlock buyers and—ultimately—the larger enterprise market. In the next section, we’ll explore the three most promising spaces where these startups will build.
Three areas of opportunity where startups can win
It’s not easy for startups to compete in a market that favors established players. But where startups have an advantage is in their ability to blaze new trails in areas that incumbents may initially disregard or dismiss, and their willingness to embrace markets so new that they’re anybody’s game.
We’ve identified three areas that offer enormous potential for startups to break out:
- Vertical AI. In industry-specific applications, AI will reinvent human-machine collaboration, becoming the driver for end-to-end automation rather than merely a copilot or collaboration platform.
- Horizontal AI. Horizontal solutions are popular because they can be used across industries and departments, increasing workflow efficiency beyond what was previously possible. As AI becomes more capable of reasoning, collaborating, communicating, learning, and predicting, next-generation workflow tools will not only allow machines to augment or automate routine tasks, but—with advanced approaches like agents and multi-step reasoning—take on work that only humans could do before.
- The modern AI stack. New generative capabilities require new tools for building LLM apps, including databases, serving infrastructure, data orchestration, and pipelines. Although still coalescing, the modern AI stack attracts the most significant percentage of enterprise AI investment, making it the largest market in the generative AI domain and an attractive focus for startups.
Vertical AI
The incredible rise of vertical SaaS in recent years has demonstrated the demand for software tailored to the needs of specialized industries and given rise to companies like Toast (restaurant POS), Procore (construction), and Benchling* (life sciences).
But when generative AI is introduced, industry-specific tools gain superpowers. Workflows are optimized, tasks automated, and the user experience tailored not at the industry level but even more granularly—to organization or even individual needs and preferences.
The emerging AI medical scribe market provides an example for this. Rather than requiring doctors to manually chart while they see patients, next-generation solutions like Eleos Health* leverage valuable industry-specific data and reinforcement learning to tailor medical notes to the guidelines and styles of each institution—and eventually, each provider.
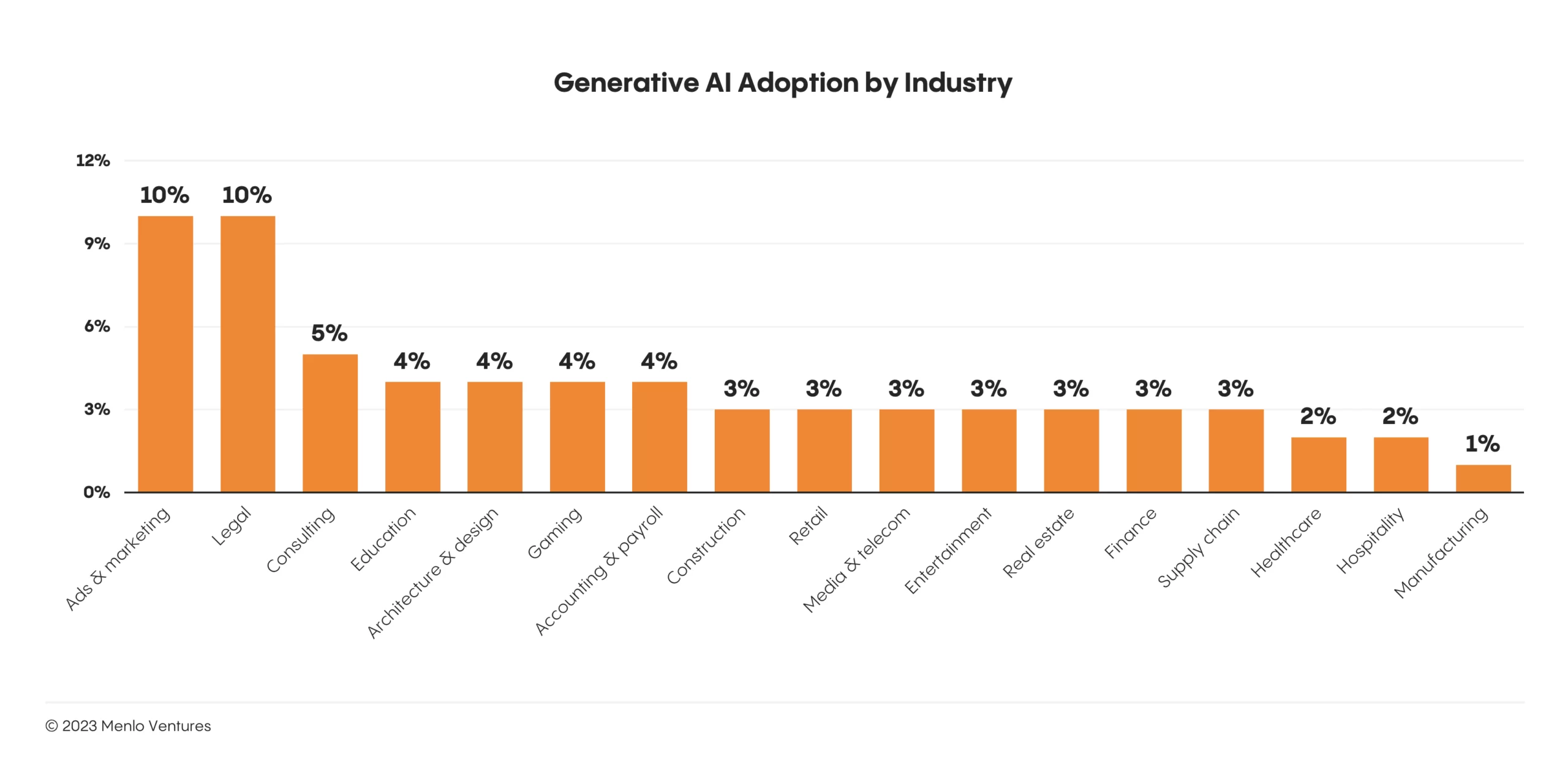
Our research revealed that two sectors are leading the way for vertical generative AI adoption: marketing and legal. As an example, marketers have embraced Synthesia, an AI tool that makes it easy to quickly produce high-quality video content. Meanwhile, law firms have turned to Eve* and Harvey to take on the labor-intensive work of contract analysis, due diligence, litigation, and regulatory compliance.
Over time, we will see more conservative industries, like healthcare and finance, embracing the value of generative AI too. Although executives in these industries report fewer use cases for generative AI today, our survey reveals that AI is a top roadmap priority.
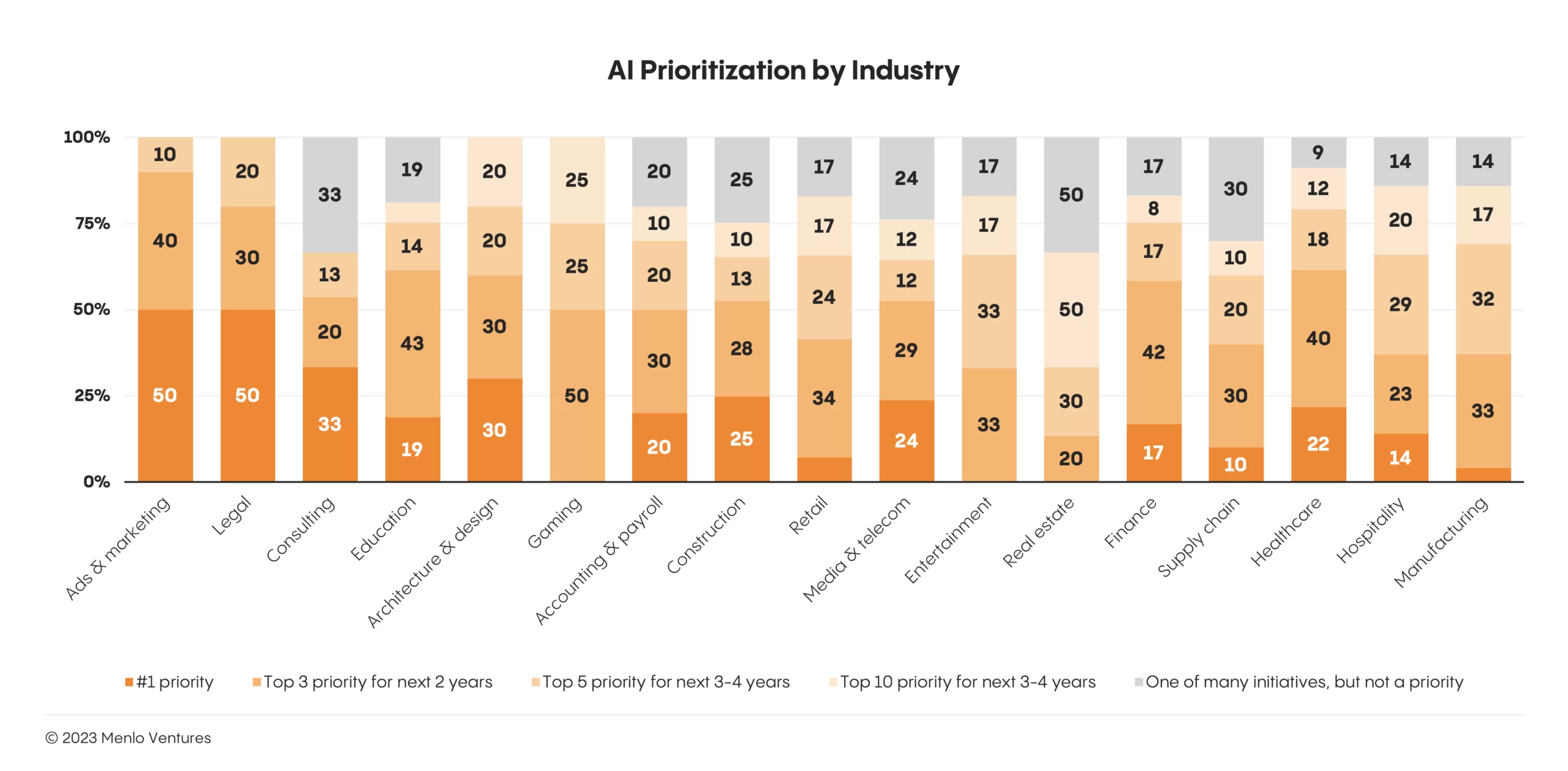
Many are currently piloting automation solutions like AI clinical workflow automation (e.g., Co:Helm, Latent) and copilots for financial analysis (e.g., Minerva). The market map below highlights a few of the most promising early vertical AI startups.
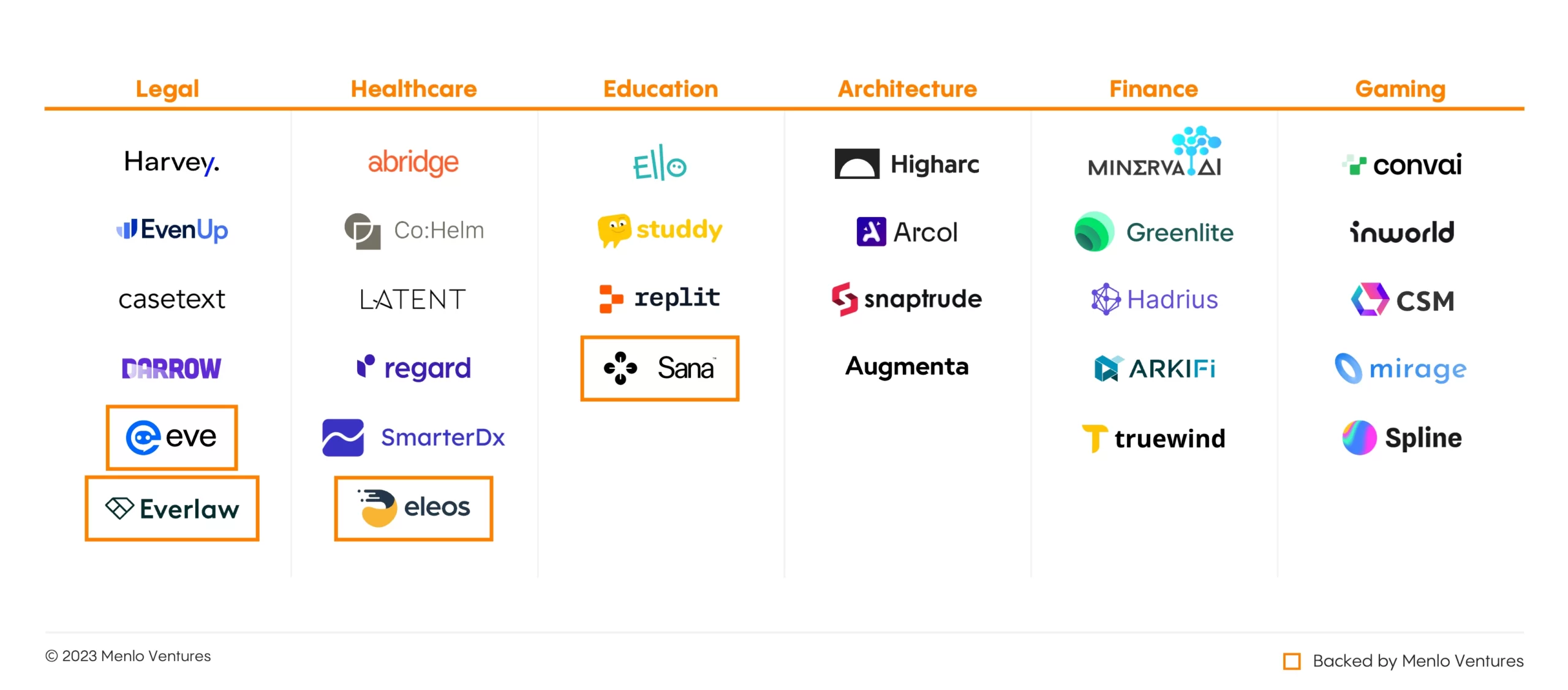
Horizontal AI
We also see opportunities for startups to win with horizontal solutions that use AI to superpower employees by automating away manual tasks. A growing wave of horizontal software will enable AI-powered use cases across departments, spanning workflow automation, internal tools/applications, and next-gen RPA.
While the previous generation of horizontal automation players (e.g., Zapier, Retool, UiPath) are undergoing an AI retrofit, A new wave of AI-native challengers—including Adept and Lindy*—combine foundation model intelligence and bespoke models trained on click and UI data to deliver autonomy their predecessors could not touch. Their ability to reason dynamically (versus follow a rigid, fallible set of programmed steps to complete a task) unlocks enormous potential.
Beyond workflow automation, we anticipate the rise of agents that think and act independently. Sophisticated personal agents will span the workday—handling emails, calendars, note-taking, and more—and move into department- and domain-specific workflows. Like an executive assistant, give them a job to do (e.g., update a series of CRM entries, provide background on meeting attendees and document next steps) and they will execute on your behalf. AI agents gain intelligence over time and, as advanced reasoning technology (e.g., chain-of-thought, tree-of-thought, and reflexion) matures, employees will be able to reliably offload many of their repetitive and manual tasks to these agents.
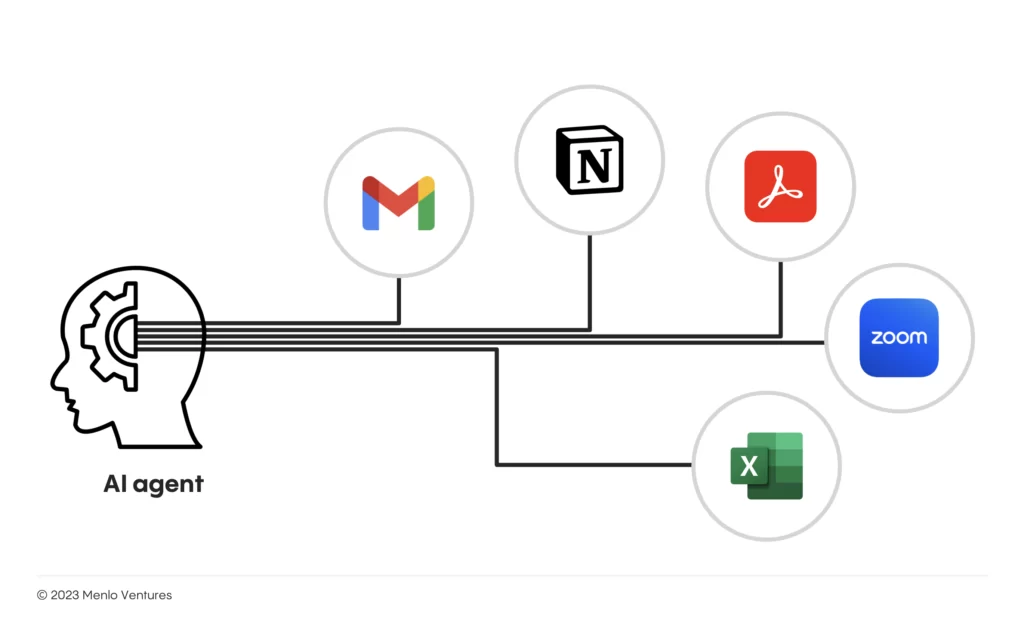
It’s not hard to imagine that, someday, AI will have access to every spoken word and mouse click—and we like it. AI could have an incredible, immediate, and obvious impact on productivity if given access to an entire corpus of work (every call, meeting, email, note, browser search, etc). Today, we feel the tension between sacrificing privacy and gaining productivity, but this tradeoff will become easy as AI gains trust. This shift is already underway; we see discrete products used for specific use cases such as using Gong or Zoom AI Companion to record sales calls. If similar solutions can deliver value and increase productivity, these “observation for optimization” tools will ride shotgun as we work, making us smarter and better at our jobs.
Just as SOC-2 became the gold standard of compliance in the first SaaS wave, the ecosystem will converge around security requirements that enable our AI future. Legislation and corporate policy will have to evolve, but we believe that with proper RBAC,5 governance, security, and private access to an enterprise’s knowledge repository (email, documents, presentations, and more), AI can provide valuable automation and function as a natural extension of the human team.
As AI becomes more sophisticated, we will increasingly rely on these tools to make us faster, smarter, and better at our jobs. AI will lose its novelty and become an unsurprising, if not expected, collaborator throughout the workday.
The modern AI stack
Enterprises invested $1.1 billion in the modern AI stack this year, making it the largest new market in the generative AI domain and an attractive focus for startups.
The enterprise buyers we polled report that 35% of their infrastructure dollars go to foundation models like OpenAI and Anthropic*. They spend even more on the data infrastructure around these models ($650 million).
In the first half of the year, the modern AI stack was the Wild West. It was under constant construction and revision, making it difficult for buyers to know where to invest.
In the last six months, the industry has converged around some core components and standard practices for enterprise deployment, providing a higher degree of stability and standardization.
Our research revealed:
- Most models are off-the-shelf. Only 10% of enterprises pre-train their own models.
- Closed-source models like those from Anthropic and OpenAI dominate—comprising upwards of 85% of models in production—compared to open-source options like Llama 2 and Mistral.
- 60% of enterprises adopt multiple models (and route prompts to the most performant model). This multi-model approach eliminates single-model dependency, offers higher controllability, and cuts costs.
- 96% of spend today is directed towards inference, indicating that more budget goes to running models vs. training them where the need is more episodic.
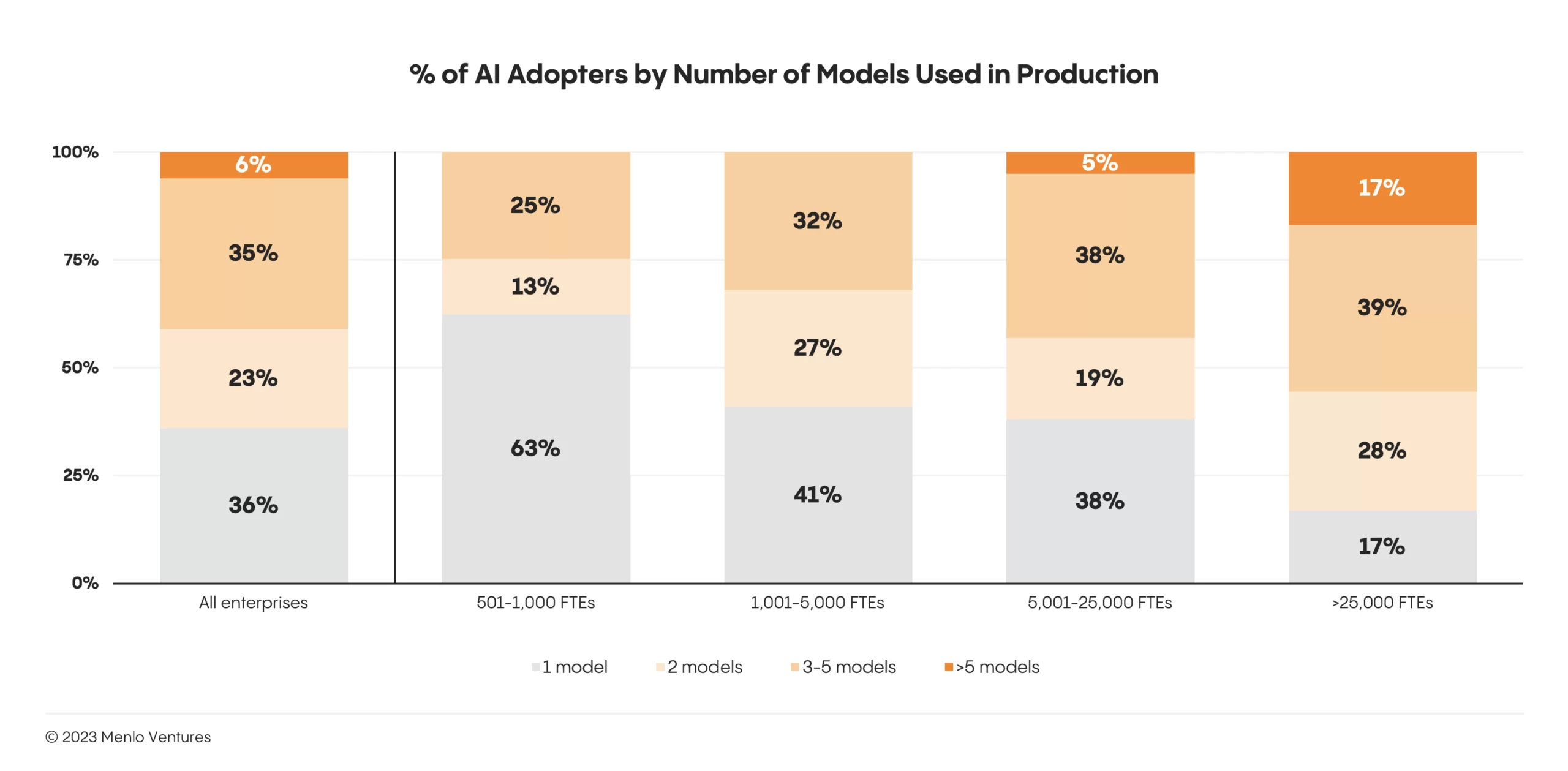
- Middleware and tooling are still developing areas. Prompt engineering is the most popular customization method, while the most popular evaluation method is human review.
- Retrieval-augmented generation (RAG) is becoming a standard. RAG is an AI framework that augments LLMs with relevant and current information retrieved from external knowledge bases to improve the quality of responses. This dynamic augmentation allows LLMs to overcome the limitations of fixed datasets to generate responses that are up-to-date and contextually relevant. Thirty-one percent of AI adopters we surveyed are using this approach, more than those fine-tuning (19%), implementing adapters (18%), or leveraging RLHF (13%).6
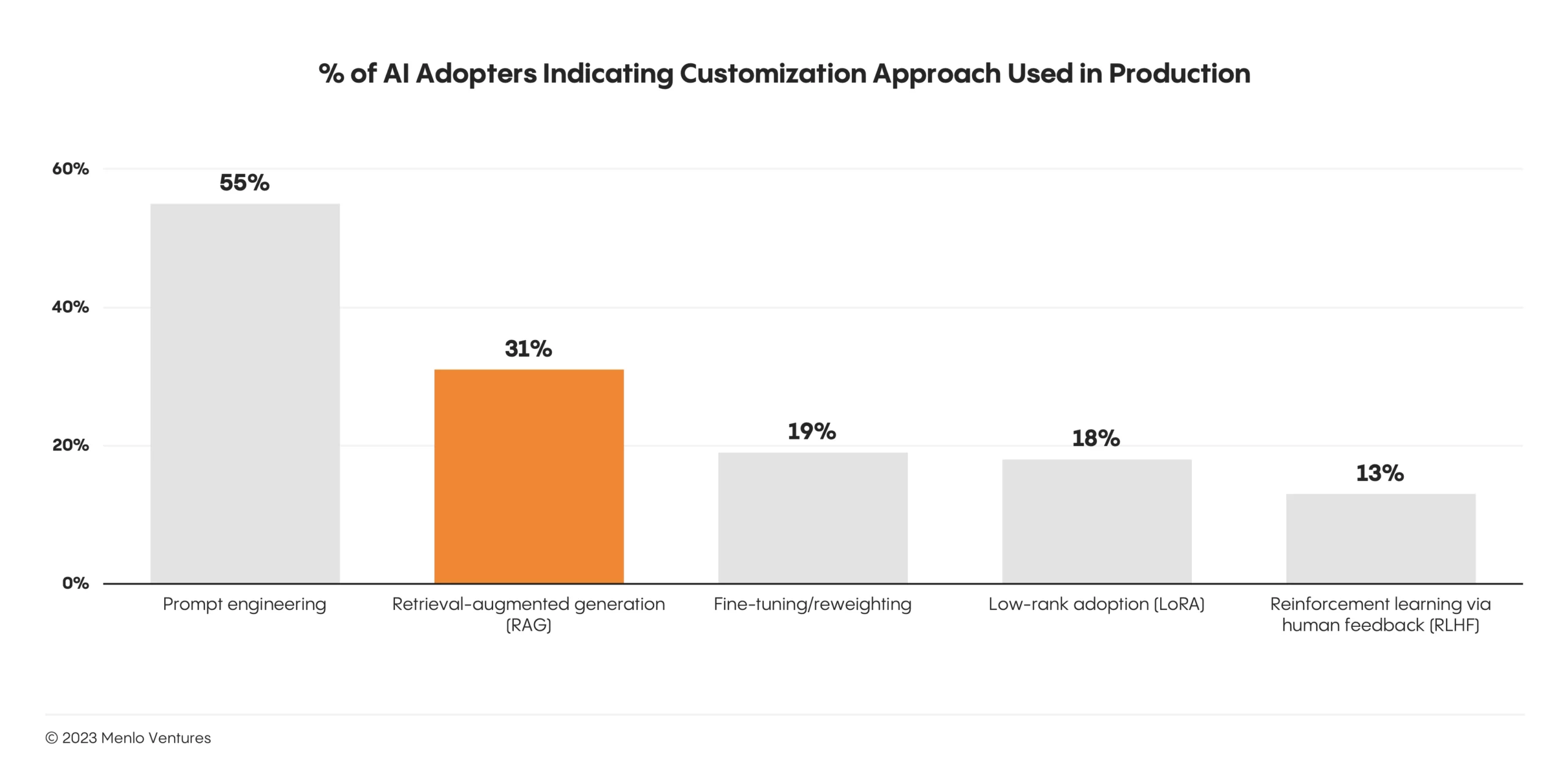
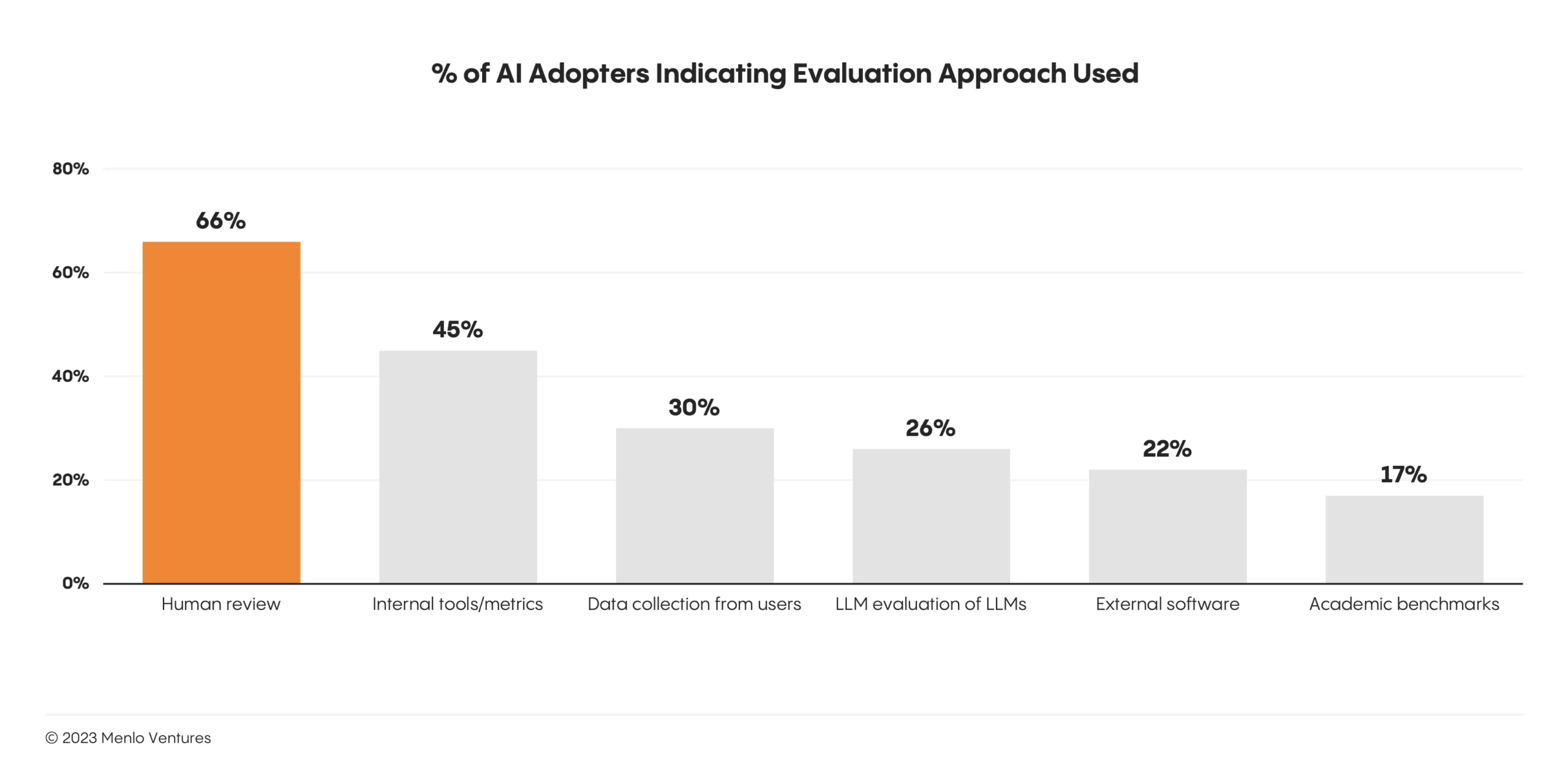
Despite having standardized around some core components and approaches, the modern AI stack is by no means settled. As this year proved, it will continue to evolve: Many of today’s prominent players, including Scale, HuggingFace, and Weights & Biases, were first built for traditional MLOps, but found renewed relevance in the modern AI stack. We’ve also seen new players emerge, like Pinecone*, which scaled with the popularization of RAG.
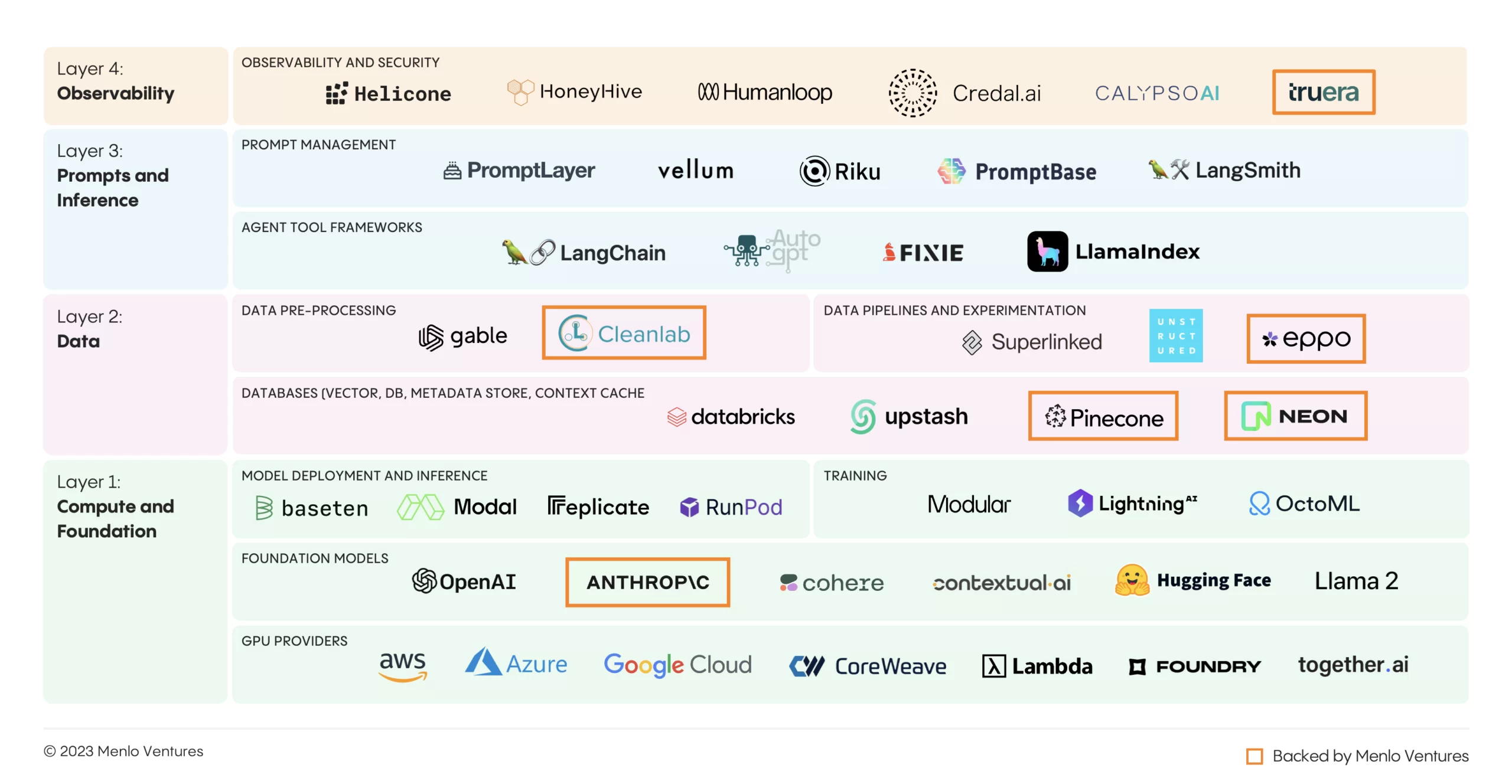
New building blocks will continue to rise in prominence, creating an incredible opportunity for startups. We are most excited about these emerging areas of the stack.
- Model deployment and inference. Like the modern data stack, the modern AI stack will be serverless, freeing developers from the complexity of running applications, facilitating rapid iteration, and optimizing resources, so that enterprises pay for compute versus availability.
Already, companies like Baseten and Modal offer serverless remote environments to run and deploy models, enabling unparalleled performance and cost efficiency while minimizing the pain of manual deployment and inference—including manually configuring Kubernetes, server provisioning, permissioning, and autoscaling. - Data transformations and pipelines. Data must be pre-processed before it hits the LLM. This includes extracting relevant context from data stores across the organization, transforming the data into a usable format, and loading the data into the model’s context window or a vector database for retrieval—with the immediate response time users have come to expect when prompting an LLM.
Unstructured and Superlinked are emerging as leading “ETL for LLMs”7—handling the intricate data pipeline creation for incoming batch and streaming data sources, managing embeddings, and enabling real-time synchronization so enterprise LLMs always have the most up-to-date context across user and content models. - Observability and security. Proper data governance and LLM security will be critical to enterprise deployment. Domain specialists like Credal (data loss prevention), Calypso (content governance), and HiddenLayer (threat detection and response) allow companies to securely connect internal data stores to third-party foundation models or customer-facing AI applications—ensuring full transparency, auditability, and traceability.
What’s next?
2023, the year of AI hype, is giving way to the hard work of real AI adoption in 2024.
Although the market is still early today and dominated by incumbents offering incremental innovations, the opportunity is emerging for startups to take the lead and innovate at the forefront of this next era of computing history. Emergent properties in reasoning and creation—enabled by next-generation techniques like agents, chain-of-thought reasoning, and reflexion—will propel the next wave of generative AI-native players to rewrite enterprise workflows and create new greenfield markets.
The team at Menlo is eager to partner with forward-thinking founders who are seeking to upend the status quo and lead the charge. Already, we’ve invested in some of the most transformative companies in the space—including Anthropic, Pinecone, Aisera, Typeface, Cleanlab, Sana Labs, Eleos Health, Lindy, and Eppo.
There has never been a better time to build in generative AI. At Menlo, we’re incredibly optimistic about what is to come for generative AI in the enterprise. If you are a founder building across the generative AI stack, we’d love to chat with you.
*Backed by Menlo Ventures
- Estimates based on Menlo Ventures’ 2023 Enterprise AI survey (N=453) and a bottoms-up revenue analysis
- Estimates based on Menlo Ventures’ 2023 Enterprise AI survey (N=453) and a bottoms-up revenue analysis
- Internet penetration via World Bank; mobile penetration via Morgan Stanley Research; cloud penetration via CapIQ
- The Information
- Role-based access control (RBAC) is the concept of securely managing access by assigning and restricting user access based on clearly established roles.
- Reinforcement learning from human feedback (RLHF) is a machine learning approach that combines reinforcement learning techniques, such as rewards and comparisons, with human guidance to train an artificial intelligence (AI) agent.
- ETL stands for extract, transform, and load. This is the process data engineers use to take data from various sources, transform the data into a usable and trustworthy resource, and load that data into a central repository that end users can access.
Naomi is a venture partner at Menlo Ventures focused on AI-enabled SaaS companies innovating across workflow automation, data/developer-first tooling, and productivity. She looks for platforms leveraging AI to bring efficiency to businesses—helping automate the mundane and painful parts of peoples’ jobs, freeing them up to focus on higher-order problems. She’s…
As an investor at Menlo Ventures, Derek concentrates on identifying investment opportunities across the firm’s thesis areas, covering AI, cloud infrastructure, digital health, and enterprise SaaS. Derek is especially focused on investing in soon-to-be breakout companies at the inflection stage. Derek joined Menlo from Bain & Company where he worked…