OpenAI’s ChatGPT reached 100 million monthly active users just two months after launch, setting the new standard for the fastest-growing consumer application in history. The landmark achievement underscored the strength of generative AI’s pull in consumer markets—surpassing even the breakthroughs of the internet and smartphones.
Both B2C startups and incumbents will now have to grapple with how they will play in the new age of AI.
Things are moving fast, and nobody wants to be left behind. Leading software companies like Microsoft and Salesforce are already building AI functionalities into their products. At the same time, early-stage startups are bringing AI technology to every software vertical. (The latest batch of Y Combinator startups included so many generative AI companies that they could form their own market map.)
Now is the time to build. Winners in these markets will create billions of dollars of economic value and deliver consumer experiences bordering on magic. Those left behind risk going the way of strip malls, paper maps, and phone books.
In this article, we’ll break down some of the key AI building blocks that consumer companies will use to drive the next wave of innovation.
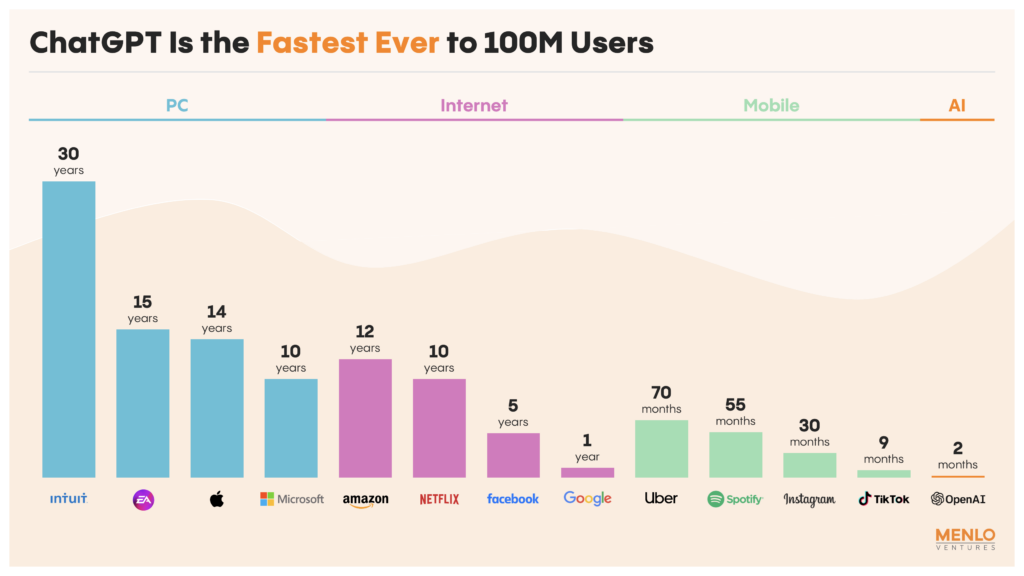
The Building Blocks
AI primitives, in both their closed-source and open-source flavors, are available for companies to use like Lego blocks to build category-defining products that were simply not possible before.
At the foundation of the recent emergence of generative AI ingredients is the transformer deep learning architecture. These models make numeric representations of text, images, and audio via embeddings, capturing the relationships between different types of inputs with equations that allow software to “understand” complex, multi-modal data such as human language, image composition, or speech.
When pre-trained on larger and larger datasets, they can map relationships between concepts and become exponentially more powerful—demonstrating emergent capabilities with each new generation of models, including creative generation, summarization, and categorization; complex reasoning; and reasoning with knowledge.
A look at the progress of the GPT family demonstrates the growth. Between GPT-1 (2018) and GPT-4 (2023), AI moved from having the language capabilities of a toddler to earning top marks on graduate and professional-level tests.
The transformer architecture has since proliferated across modalities (text, image, video, audio, etc.)—creating powerful primitives with which companies can build.
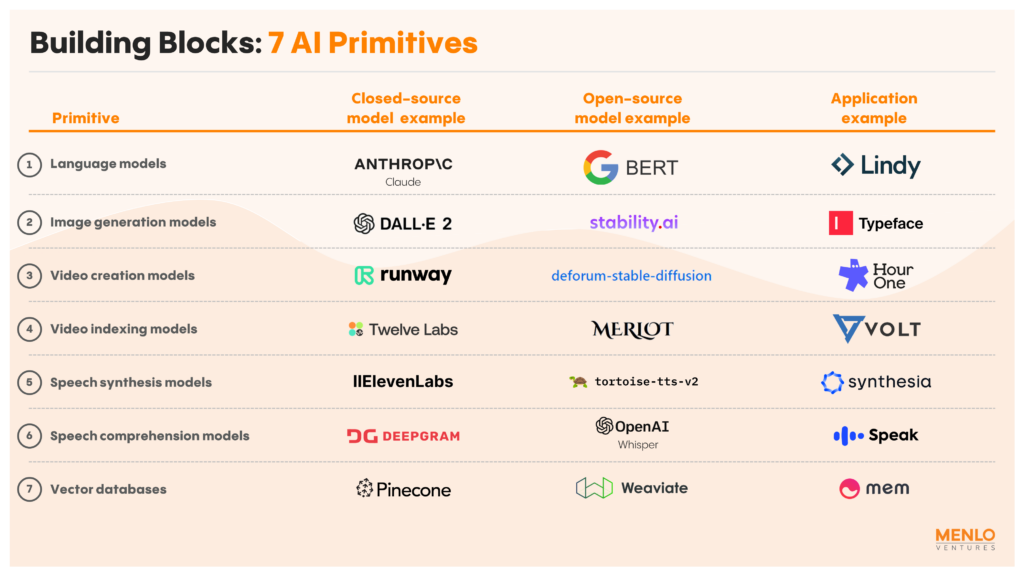
The seven most important generative AI primitives today are:
Language Models
Pre-trained transformer models like OpenAI’s GPT and Anthropic’s Claude improve on the domain-specific NLP models that dominated the last decade. These new models outperform the average human across a broad spectrum of complex language tasks such as reading comprehension, standardized test-taking, coding, and more. Many can be further customized for specific horizontal use cases (e.g., marketing) or vertical ones (e.g., healthcare) with fine-tuning and embeddings. Plug-ins allowing real-time interaction with our favorite web services will compound their capabilities.
Image Generation Models
The transformer model has also revolutionized new image creation. Pioneering models like OpenAI’s CLIP in 2021 leveraged vision transformers to improve text-to-image capabilities, while new techniques like image diffusion (e.g., as applied by DALL-E 2 and Stable Diffusion) have enabled AI to create images that are now more hyperrealistic, high-definition, flexible, controllable, and coherent than ever before.
Video Creation Models
Video generation is still a step behind image generation when it comes to matching the best image models’ studio-grade quality. However, RunwayML’s Gen-2 model is making tremendous strides in the area. The model employs a latent diffusion architecture that is both structure and content-aware, allowing it to understand the relationship between objects that appear from one frame to the next and coherently depict them in a shot. This represents a notable advancement over previous approaches to video generation, which have more awkwardly tried to stretch image generation model techniques to video by computing explicit correspondences between objects in separate still-image frames or fine-tuning on each individual video.
Video and Image Indexing Models
An enormous amount of unstructured data is locked away in image and video today due to technical barriers posed by context understanding. First-generation image labeling models like Clarifai* first changed this via models for object identification, image classification, and semantic segmentation. More recently, transformer-based models like Twelve Labs’ ViSeRet are extending these capabilities to video—making video content searchable for objects and people, movement and actions, dialogue and sound, and on-screen text.
Speech Synthesis Models
Speech synthesis models come in both text-to-speech (TTS) and voice-to-voice variations. In TTS, models like those developed by Wellsaid, Suno, and Eleven Labs modify speech waveforms to create human-like qualities, including nuanced intonations, differing cadences, and highly controllable expressiveness. Voice-to-voice applies many of the same technologies but in real time—with some leading models like Voice.ai and Sanas boasting only 400 ms of delay (compared to 60-70 ms, which is imperceptible to most people over video).
Speech Comprehension Models
Automatic speech recognition (ASR) is the process of converting spoken language into text. Models in this category, like Google Speech-to-Text, have been around for years. More recently, transformer models like OpenAI’s Whisper—pre-trained on large datasets of multilingual and multitask supervised data collected from the web—have started to outperform these existing models, with more robustness to accents, background noise, and technical language.
Vector Databases
Today’s language, image, and speech models are highly performant in few- and zero-shot learning scenarios but still fall short of the level of personalization that consumers expect. For this last mile, the semantic clustering of similar items with tools like Pinecone* provides an important solution. Vector databases allow users to store unstructured assets like writings and images as vectors, the common data format used in many AI applications. In this way, startups can augment their AI models’ knowledge with their and customers’ data for better, more personalized experiences.
Building With AI
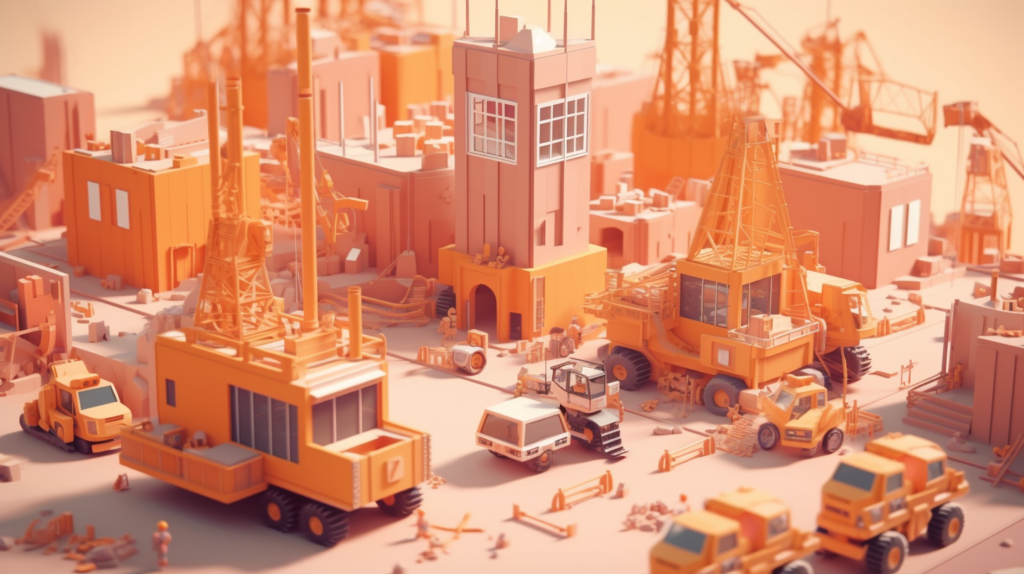
These new AI primitives—together with the continued improvement of earlier AI capabilities including collaborative filtering and content filtering (e.g., TikTok); machine vision (e.g., OpenSpace*); and pattern recognition and categorization—comprise a comprehensive palette of ingredients that will be combined in coming months and years to deliver on countless enterprise and consumer jobs better, faster, and cheaper.
To get started quickly, companies may take some of these new AI ingredient technologies off-the-shelf to power compelling new product features with minimal lift (e.g., Notion AI). Closed-source model providers like Anthropic allow builders to leverage their models with a single API call.
To build something more core to a company’s differentiation or central value proposition, organizations may want to take the longer route—customizing open-source versions like Stable Diffusion for image generation or tortoise-tts for voice synthesis. This allows for more programmatic, modular control and better performance, cost, and security but also requires a certain level of ML sophistication and resources and risks falling behind as the market-leading models continue to improve.
Already, we see entrepreneurs leveraging these tools with both approaches for powerful results. Let’s take a look at two promising examples:
Synthesia. The AI avatar company’s mission is to make video creation easy. Using Synthesia’s platform, customers from Amazon to Reuters have generated lifelike synthetic humans for training, how-to videos, and marketing directly from a script in a process the company calls text-to-video. If that sounds a tad too fantastical, that’s because it was inconceivable until very recently. Previous attempts have resulted in AI avatars that came off more like Ex Machina than Westworld. But now, Synthesia can cross the uncanny valley with the introduction of the following AI primitives:
- Language models. Corporate learning & development videos usually start with a script. Users can upload their own, or—for those that want a quick start—Synthesia integrates with GPT-4 so that users can have a script generated for them with the touch of a button.
- Speech synthesis. Audio is the next important step in video synthesis. Here Synthesia leverages the lifelike voice generators of third-party solutions, including Wellsaid and Eleven Labs, to create human-sounding speech that simply wasn’t possible a year or two prior.
- Video creation models. Synthesia’s secret sauce is its ability to generate lifelike synthetic humans that look, act, and sound exactly like an actual person would—with proprietary video synthesis models that can create lifelike avatars with remarkably human-looking facial expressions and nonverbal cues.
Typeface. Typeface* is a Menlo Ventures-backed company with an enterprise-grade generative AI application that drives high-quality, brand-personalized content development at speed and scale. With Typeface, a marketer can type in commands like, “Write me a blog post about the new product we’re launching in Asia” and have the platform execute it, writing several paragraphs about the product launch in the brand’s voice, along with accompanying media that can be targeted toward specific demographics. The company leverages several new AI primitives to do this:
- Image generation. At the core of Typeface’s solution is a personalized AI core that initially leveraged Stable Diffusion’s open-source image generation model. On top of this, the company then built a proprietary Affinity AI model that self-trains and customizes content to each brand’s unique voice, style, and workflows, unifying content velocity with brand personalization and control.
- Language models. To build an end-to-end platform solution for creative professionals, Typeface wrapped GPT-4 with the same Affinity AI model and workflow and collaboration features. This architecture allows users to access a fine-tuned version of the large language model but bring their brand’s unique data, voice, and style to the platform through a continuously-learning feedback cycle.
What’s Next
The age of AI is now upon us. The powerful new primitives available to builders and the products they enable will fundamentally change how we live, work, and play. And more AI primitives are coming: From language to motion and animation, 3D asset creation, human-like interaction with computer UIs, and more. The mixing and matching of these primitives, applied to different domains, will prove nothing short of mind-blowing as AI emerges as the driving force in a coming decade of innovation.
At Menlo Ventures, we’ve been investing in and tracking AI for years. We’ve already made several investments in the space, dating back to our 2008 Series A investment in Siri, the AI voice assistant. More recently, Menlo has made investments in vector databases (Pinecone), explainable AI (TruEra), productivity (Lindy), corporate content generation (Sana Labs), and creative workflows (Typeface).
But as our overview of today’s market indicates, we are still in the early innings. We expect that AI will become a necessary ingredient for the next wave of innovators. (You can read more about the power of ingredient technologies here in an article Shawn wrote for TechCrunch.) Most of the generational companies that will emerge have yet to be built. In subsequent articles, we’ll dive deeper into some of the exciting consumer categories we hope to see entrepreneurs transform with the power of AI.
In the meantime, we’re excited to hear from entrepreneurs who see the potential for AI to address consumers’ most pressing needs. If this sounds like you, shoot us a note. We’d love to hear from you:
Shawn Carolan: [email protected]
Derek Xiao: [email protected]
This article was written with the help of generative AI solutions from Anthropic and Typeface, with some accompanying graphics produced by Midjourney. When we were done, we checked our work with Grammarly.
* Stars denote Menlo Ventures portfolio companies.
As an early-stage investor, Shawn focuses on companies that serve the “utilitarian consumer”—the individual seeking better, faster, and cheaper ways to move through life. Because basic human needs are persistent, he looks at how people are spending their money and time to assess the value and utility of a product…
As an investor at Menlo Ventures, Derek concentrates on identifying investment opportunities across the firm’s thesis areas, covering AI, cloud infrastructure, digital health, and enterprise SaaS. Derek is especially focused on investing in soon-to-be breakout companies at the inflection stage. Derek joined Menlo from Bain & Company where he worked…